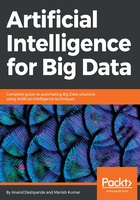
Frequently asked questions
Let's have a small recap of the chapter:
Q: What are Ontologies and what is their significance in intelligent systems?
A: Ontology as a generic term means the knowledge of everything that exists in this universe. As applicable to information systems, Ontologies represent a semantic and standardized view of the world's knowledge assets. They are domain-specific representations of knowledge and models related to real world entity representations. The intelligent systems that link heterogeneous knowledge domains need to have access to consistent representations of knowledge in order to interoperate and understand contextual events to make inferences and decisions, which trigger actions and hence results, in order to complement human capabilities.
Q: What are the generic properties of Ontologies?
A: Ontologies should be complete, unambiguous, domain-specific, generic, and extensible.
Q: What are the various components of Ontologies?
A: Various Ontology components are Concepts, Slots, Relationships, Axioms, Instances, and Operations.
Q: What is the significance of a universal data format in knowledge management systems?
A: The Resource Description Format (RDF) intends to be the universal format for knowledge representation, allowing heterogeneous systems to interact and integrate in a consistent and reliable manner. This forms the basis of the semantic view of the world.
Q: How is it possible to model the worldview with Ontologies? Is it possible to automate the Ontology definition process considering vast and ever-increasing knowledge stores in the universe?
A: Knowledge assets are growing exponentially in size with time. In order to create an Ontological representation of these assets, we need an automated approach, without which it will be difficult to catch up with the volume. Ontology learning takes an algorithmic approach by leveraging distributed computing frameworks to create a baseline model of the worldview. The Ontology learning process retrieves textual, unstructured data from heterogeneous sources, refines it, and represents it in a hierarchical manner. This is realigned with post-processing by reusing existing domain-specific knowledge assets, and finally released for generic consumption by intelligent agents.