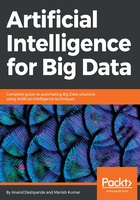
上QQ阅读APP看书,第一时间看更新
Ontology learning process
The Ontology learning process consists of six Rs:
They are explained as followed:
- Retrieve: The knowledge assets are retrieved from the web and application sources from the domain specific stores using web crawls and protocol-based application access. The domain specific terms and axioms are extracted with a calculation of TF/IDF values and by the application of the C-Value / NC Value methods. Commonly used clustering techniques are utilized and the statistical similarity measures are applied on the extracted textual representations of the knowledge assets.
- Refine: The assets are cleansed and pruned to improve signal to noise ratio. Here, an algorithmic approach is taken for refinement. In the refinement step, the terms are grouped corresponding to concepts within the knowledge assets.
- Represent: In this step, the Ontology learning system arranges the concepts in a hierarchical structure using the unsupervised clustering method (at this point, understand this as a machine learning approach for the segmentation of the data; we will cover the details of unsupervised learning algorithms in the next chapter).
- Re-align: This is a type of post-processing step that involves collaboration with the domain experts. At this point, the hierarchies are realigned for accuracy. The Ontologies are aligned with instances of concepts and corresponding attributes along with cardinality constraints (one-to-one, one-to-many, and so on). The rules for defining the syntactic structure are defined in this step.
- Reuse: In this step, similar domain-specific Ontologies with connection endpoints are reused, and synonyms are defined in order to avoid parallel representations of the same concepts, which are finalized across other Ontology definitions.
- Release: In this step, the Ontologies are released for generic use and further evolution.