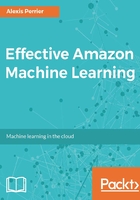
上QQ阅读APP看书,第一时间看更新
Underfitting
Underfitting means that the model was poorly trained. Either the training dataset did not have enough information to infer strong predictions, or the algorithm that trained the model on the training dataset was not adequate for the context. The algorithm was not well parameterized or simply inadequate for the data.
If we measure the prediction error not only on the validation set but also on the training set, the prediction error will be large if the model is underfitting. Which makes sense: if the model cannot predict the training, it won't be able to predict the outcomes in the validation set it has not seen before. Underfitting basically means your model is not working.
Common strategies to palliate this problem include:
- Getting more data samples – If the problem comes from a dataset that is too small or does not contain sufficient information, getting more data may improve the model performance.
- Adding more features, raw or via feature engineering – by taking the log, squaring, binning, using splines or power functions. Adding many features and seeing how that improves the predictions.
- Choosing another model – Support Vector Machine, Random Forest, Boosted trees, Bayes classifiers all have different strengths in different contexts.