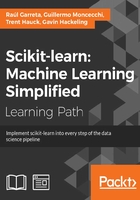
Preface
Suppose you want to predict whether tomorrow will be a sunny or rainy day. You can develop an algorithm that is based on the current weather and your meteorological knowledge using a rather complicated set of rules to return the desired prediction. Now suppose that you have a record of the day-by-day weather conditions for the last five years, and you find that every time you had two sunny days in a row, the following day also happened to be a sunny one. Your algorithm could generalize this and predict that tomorrow will be a sunny day since the sun reigned today and yesterday. This algorithm is a pretty simple example of learning from experience. This is what Machine Learning is all about: algorithms that learn from the available data.
This course is designed in the same way that many data science and analytics projects play out. First, we need to acquire data; the data is often messy, incomplete, or not correct in some way. Therefore, we spend the first chapter talking about strategies for dealing with bad data and ways to deal with other problems that arise from data. For example, what happens if we have too many features? How do we handle that?
What this learning path covers
Module 1, Learning scikit-learn: Machine Learning in Python, in this module, you will learn several methods for building Machine Learning applications that solve different real-world tasks, from document classification to image recognition. We will use Python, a simple, popular, and widely used programming language, and scikit-learn, an open source Machine Learning library. In each chapter of this module, we will present a different Machine Learning setting and a couple of well-studied methods as well as show step-by-step examples that use Python and scikit-learn to solve concrete tasks. We will also show you tips and tricks to improve algorithm performance, both from the accuracy and computational cost point of views.
Module 2, scikit-learn Cookbook, the first chapter of this module is your guide. The meat of this module will walk you through various algorithms and how to implement them into your workflow. And finally, we'll end with the postmodel workflow. This chapter is fairly agnostic to the other chapters of the module and can be applied to the various algorithms you'll learn up until the final chapter.
Module 3, Mastering Machine Learning with scikit-learn, in this module, we will examine several machine learning models and learning algorithms. We will discuss tasks that machine learning is commonly applied to, and learn to measure the performance of machine learning systems. We will work with a popular library for the Python programming language called scikit-learn, which has assembled excellent implementations of many machine learning models and algorithms under a simple yet versatile API.
This module is motivated by two goals:
- Its content should be accessible. The book only assumes familiarity with basic programming and math.
- Its content should be practical. This book offers hands-on examples that readers can adapt to problems in the real world.
What you need for this learning path
Module 1:
For running the module's examples, you will need a running Python environment, including the scikit-learn library and NumPy and SciPy mathematical libraries. The source code will be available in the form of IPython notebooks. For Chapter 4, Advanced Features, we will also include the Pandas Python library. Chapter 1, Machine Learning – A Gentle Introduction, shows how to install them in your operating system.
Module 2:
Here are the contents that will get the environment set up. This will allow you to follow along with the code in this module. This method may be easier for less-experienced Python developers:
dateutil==2.1
ipython==2.2.0
ipython-notebook==2.1.0
jinja2==2.7.3
markupsafe==0.18
matplotlib==1.3.1
numpy==1.8.1
patsy==0.3.0
pandas==0.14.1
pip==1.5.6
pydot==1.0.28
pyparsing==1.5.6
pytz==2014.4
pyzmq==14.3.1
scikit-learn==0.15.0
scipy==0.14.0
setuptools==3.6
six==1.7.3
ssl_match_hostname==3.4.0.2
tornado==3.2.2
Module 3:
The examples in this module assume that you have an installation of Python 2.7. The first chapter will describe methods to install scikit-learn 0.15.2, its dependencies, and other libraries on Linux, OS X, and Windows.
Who this learning path is for
If you are a programmer and want to explore machine learning and data-based methods to build intelligent applications and enhance your programming skills, this is the book for you. No previous experience with machine-learning algorithms is required.
Reader feedback
Feedback from our readers is always welcome. Let us know what you think about this course—what you liked or disliked. Reader feedback is important for us as it helps us develop titles that you will really get the most out of.
To send us general feedback, simply e-mail <feedback@packtpub.com>
, and mention the course's title in the subject of your message.
If there is a topic that you have expertise in and you are interested in either writing or contributing to a book, see our author guide at www.packtpub.com/authors.
Customer support
Now that you are the proud owner of a Packt course, we have a number of things to help you to get the most from your purchase.
Downloading the example code
You can download the example code files for this course from your account at http://www.packtpub.com. If you purchased this course elsewhere, you can visit http://www.packtpub.com/support and register to have the files e-mailed directly to you.
You can download the code files by following these steps:
- Log in or register to our website using your e-mail address and password.
- Hover the mouse pointer on the SUPPORT tab at the top.
- Click on Code Downloads & Errata.
- Enter the name of the course in the Search box.
- Select the course for which you're looking to download the code files.
- Choose from the drop-down menu where you purchased this course from.
- Click on Code Download.
You can also download the code files by clicking on the Code Files button on the course's webpage at the Packt Publishing website. This page can be accessed by entering the course's name in the Search box. Please note that you need to be logged in to your Packt account.
Once the file is downloaded, please make sure that you unzip or extract the folder using the latest version of:
- WinRAR / 7-Zip for Windows
- Zipeg / iZip / UnRarX for Mac
- 7-Zip / PeaZip for Linux
The code bundle for the course is also hosted on GitHub at https://github.com/PacktPublishing/scikit-learn-Machine-Learning-Simplified. We also have other code bundles from our rich catalog of books, videos, and courses available at https://github.com/PacktPublishing/. Check them out!
Errata
Although we have taken every care to ensure the accuracy of our content, mistakes do happen. If you find a mistake in one of our courses—maybe a mistake in the text or the code—we would be grateful if you could report this to us. By doing so, you can save other readers from frustration and help us improve subsequent versions of this course. If you find any errata, please report them by visiting http://www.packtpub.com/submit-errata, selecting your course, clicking on the Errata Submission Form link, and entering the details of your errata. Once your errata are verified, your submission will be accepted and the errata will be uploaded to our website or added to any list of existing errata under the Errata section of that title.
To view the previously submitted errata, go to https://www.packtpub.com/books/content/support and enter the name of the course in the search field. The required information will appear under the Errata section.
Piracy
Piracy of copyrighted material on the Internet is an ongoing problem across all media. At Packt, we take the protection of our copyright and licenses very seriously. If you come across any illegal copies of our works in any form on the Internet, please provide us with the location address or website name immediately so that we can pursue a remedy.
Please contact us at <copyright@packtpub.com>
with a link to the suspected pirated material.
We appreciate your help in protecting our authors and our ability to bring you valuable content.
Questions
If you have a problem with any aspect of this course, you can contact us at <questions@packtpub.com>
, and we will do our best to address the problem.