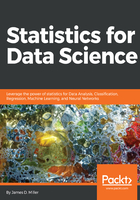
Analyzing the data and/or applying machine learning to the data
In this phase, quite a bit of analysis takes place as the data scientist (driven by a high level of scientific curiosity and experience) attempts to shape a story based upon an observation or the interpretation of their understanding of the data (up to this point). The data scientist continues to slice and dice the data, using analytics or BI packages—such as Tableau or Pentaho or an open source solution such as R or Python—to create a concrete data storyline. Once again, based on these analysis results, the data scientist may elect to again go back to a prior phase, pulling new data, processing and reprocessing, and creating additional visualizations. At some point, when appropriate progress has been made, the data scientist may decide that the data is at such point where data analysis can begin. Machine learning (defined further later in this chapter) has evolved over time from being more of an exercise in pattern recognition to now being defined as utilizing a selected statistical method to dig deeper, using the data and results of the analysis of this phase to learn and make a prediction, on the project data.
The ability of a data scientist to extract a quantitative result from data through machine learning and express it as something that everyone (not just other data scientists) can understand immediately is an invaluable skill, and we will talk more about this throughout this book.