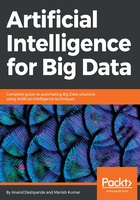
Ontology for Big Data
In the introductory chapter, we learned that big data has fueled rapid advances in the field of artificial intelligence. This is primarily because of the availability of extremely large datasets from heterogeneous sources and exponential growth in processing power due to distributed computing. It is extremely difficult to derive value from large data volumes if there is no standardization or a common language for interpreting data into information and converting information into knowledge. For example, two people who speak two different languages, and do not understand each other's languages, cannot get into a verbal conversation unless there is some translation mechanism in between. Translations and interpretations are possible only when there is a semantic meaning associated with a keyword and when grammatical rules are applied as conjunctions. As an example, here is a sentence in the English and Spanish languages:
Broadly, we can break a sentence down in the form of objects, subjects, verbs, and attributes. In this case, John and bananas are subjects. They are connected by an activity, in this case eating, and there are also attributes and contextual data—information in conjunction with the subjects and activities. Knowledge translators can be implemented in two ways:
- All-inclusive mapping: Maintaining a mapping between all sentences in one language and translations in the other language. As you can imagine, this is impossible to achieve since there are countless ways something (object, event, attributes, context) can be expressed in a language.
- Semantic view of the world: If we associate semantic meaning with every entity that we encounter in linguistic expression, a standardized semantic view of the world can act as a centralized dictionary for all the languages.
A semantic and standardized view of the world is essential if we want to implement artificial intelligence which fundamentally derives knowledge from data and utilizes the contextual knowledge for insight and meaningful actions in order to augment human capabilities. This semantic view of the world is expressed as Ontologies. In the context of this book, Ontology is defined as: a set of concepts and categories in a subject area or domain, showing their properties and the relationships between them.
In this chapter, we are going to look at the following:
- How the human brain links objects in its interpretation of the world
- The role Ontology plays in the world of Big Data
- Goals and challenges with Ontology in Big Data
- The Resource Description Framework
- The Web Ontology Language
- SPARQL, the semantic query language for the RDF
- Building Ontologies and using Ontologies to build intelligent machines
- Ontology learning