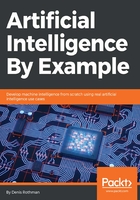
Designing datasets in natural language meetings
The reinforcement learning program described in the first chapter can solve a variety of problems involving unlabeled classification in an unsupervised decision-making process. The Q function can be applied indifferently to drone, truck, or car deliveries. It can also be applied to decision-making in games or real life.
However, in a real-life case study problem (such as defining the reward matrix in a warehouse for the AGV, for example), the difficulty will be to design a matrix that everybody agrees with.
This means many meetings with the IT department to obtain data, the SME and reinforcement learning experts. An AGV requires information coming from different sources: daily forecasts and real-time warehouse flows.
At one point, the project will be at a standstill. It is simply too complicated to get the right data for the reinforcement program. This is a real-life case study that I modified a little for confidentiality reasons.
The warehouse manages thousands of locations and hundreds of thousands of inputs and outputs. The Q function does not satisfy the requirement in itself. A small neural network is required.
In the end, through tough negotiations with both the IT department and the users, a dataset format is designed that fits the needs of the reinforcement learning program and has enough properties to satisfy the AGV.