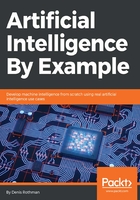
Think like a Machine
The first chapter described a reinforcement learning algorithm through the Q action-value function used by DQN. The agent was a driver. You are at the heart of DeepMind's approach to AI.
DeepMind is no doubt one of the world leaders in applied artificial intelligence. Scientific, mathematical, and applications research drives its strategy.
DeepMind was founded in 2010, was acquired by Google in 2014, and is now part of Alphabet, a collection of companies that includes Google.
One of the focuses of DeepMind is on reinforcement learning. They came up with an innovate version of reinforcement learning called DQN and referring to deep neural networks using the Q function (Bellman's equation). A seminal article published in February 2015 in Nature (see the link at the end of the chapter) shows how DQN outperformed other artificial intelligence research by becoming a human game tester itself. DQN then went on to beat human game testers.
In this chapter, the agent will be an automated guided vehicle (AGV). An AGV takes over the transport tasks in a warehouse. This case study opens promising perspectives for jobs and businesses using DQN. Thousands upon thousands of warehouses require complex reinforcement learning and customized transport optimization.
This chapter focuses on creating the reward matrix, which was the entry point of the Python example in the first chapter. To do so, it describes how to add a primitive McCulloch-Pitts neuron in TensorFlow to create an intelligent adaptive network and add an N (network) to a Q model. It's a small N that will become a feedforward neural network in Chapter 4, Become an Unconventional Innovator, and more in Chapter 12, Automated Planning and Scheduling. The goal is not to copy DQN but to use the conceptual power of the model to build a variety of solutions.
The challenge in this chapter will be to think literally like a machine. The effort is not to imitate human thinking but to beat humans with machines. This chapter will take you very far from human reasoning into the depth of machine thinking.
The following topics will be covered in this chapter:
- AGV
- The McCulloch-Pitts neuron
- Creating a reward matrix
- Logistic classifiers
- The logistic sigmoid
- The softmax function
- The one-hot function
- How to apply machine learning tools to real-life problems such as warehouse management