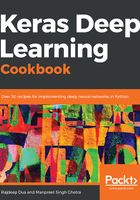
上QQ阅读APP看书,第一时间看更新
Initialize the loss
The loss is binary cross_entropy.
Cross-entropy loss, also called log loss, measures the performance of a model (classification model). The output is a probability value between 0 and 1. Cross-entropy loss increases as the predicted probability diverges from the actual value:
.

self.loss = loss or []
Initialize all internal variables for output:
self._feed_outputs = []
self._feed_output_names = []
self._feed_output_shapes = []
self._feed_loss_fns = []
Prepare the targets of the model:
self._feed_targets.append(target)
self._feed_outputs.append(self.outputs[i])
self._feed_output_names.append(name)
self._feed_output_shapes.append(shape)
self._feed_loss_fns.append(self.loss_functions[i])
Prepare sample weights:
Before compilation, the following values are assigned to sample weights and sample_weight_modes:
sample_weights = []
sample_weight_modes = []
After running through the code execution, it gets initialized to the following values:
Tensor("dense_3_sample_weights:0", shape=(?,), dtype=float32)
Prepare the metrics:
Next, we prepare metric names and metrics_tensors, which store the actual metrics:
self.metrics_names = ['loss']
self.metrics_tensors = []
Prepare total loss and metrics:
The loss is calculated and appended to self.metrics_tensors:
output_loss = weighted_loss(y_true, y_pred,
sample_weight, mask)
...
self.metrics_tensors.append(output_loss)
self.metrics_names.append(self.output_names[i] + '_loss')
Next, we calculate nested metrics and nested_weighted_metrics:
nested_metrics = collect_metrics(metrics, self.output_names)
nested_weighted_metrics = collect_metrics(weighted_metrics, self.output_names)
Initialize the test, train, and predict functions:
These are initialized lazily:
self.train_function = None
self.test_function = None
self.predict_function = None
Sort trainable weights:
In the end, we initialize the trainable weights:
trainable_weights = self.trainable_weights
self._collected_trainable_weights = trainable_weights