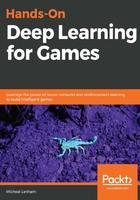
The past
The first form of a multilayer perceptron (MLP) network, or what we now call an ANN, was introduced by Alexey Ivakhnenko in 1965. Ivakhnenko waited several years before writing about the multilayer perceptron in 1971. The concept took a while to percolate and it wasn't until the 1980s that more research began. This time, image classification and speech recognition were attempted, and they failed, but progress was being made. It took another 10 years, and in the late 90s, it became popular again. So much so that ANNs made their way into some games, again, until better methods came along. Things quietened down and another decade or so passed.
Then, in 2012, Andrew Ng and Jeff Dean used an ANN to recognize cats in videos, and the interest in deep learning exploded. Their stride was one of several trivial (yet entertaining) advancements which made people sit up and take notice of deep learning. Then, in 2015, Google's DeepMind team built AlphaGo, and this time the whole world sat up. AlphaGo was shown to solidly beat the best players in the world at the game of Go, and that changed everything. Other techniques soon followed, Deep Reinforcement Learning (DRL) being one, showing that human performance could be consistently beaten in areas where that was previously not thought of as possible.