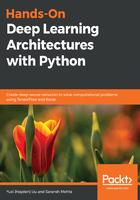
Evolutionary path to DFNs
Warren McCulloch and Walter Pitts were the first to create a model of artificial neural networks back in 1943. They built the model on something called threshold logic. A threshold was calculated by summing up inputs, and the output was binary, zero, or one, according to the threshold. In 1958, another model of a neuron was created by Rosenblatt called perceptron. Perceptron is the simplest model of an artificial neuron that can classify inputs into two classes (we discussed this neuron in Chapter 1, Getting started with Deep Learning). The concept of training neural networks by backpropagating errors using chain rule was developed by Henry J. Kelley around the early 1960s. However, backpropagation as an algorithm was unstructured and the perceptron model failed to solve that famous XOR problem. In 1986, Geoff Hinton, David Rumelhart, and Ronald Williams demonstrated that neural networks with hidden layers can learn non-linear functions with backpropagation. Further, it was also highlighted that neural networks are capable of learning any function through a universal approximation theorem. But, neural networks didn't scale to solve large problems, and by the '90s, other machine learning algorithms, such as support vector machine (SVM), dominated the space. Around 2006, Hinton once again came up with the idea of adding layers one over the other and training parameters for new layers. Deeper networks were trained using the strategy and the networks were termed as deep feedforward networks. From here, the neural networks got a new name—deep learning!
Next, we will discuss the architectural design of DFN. We will see how the layered structure is built, trained, and what significance the terms deep and feedforward carry.