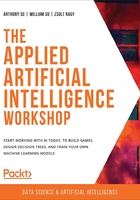
Linear Regression with One Variable
A general regression problem can be defined with the following example. Suppose we have a set of data points and we need to figure out the best fit curve to approximately fit the given data points. This curve will describe the relationship between our input variable, x, which is the data point, and the output variable, y, which is the curve.
Remember, in real life, we often have more than one input variable determining the output variable. However, linear regression with one variable will help us to understand how the input variable impacts the output variable.
Types of Regression
In this chapter, we will work with regression on the two-dimensional plane. This means that our data points are two-dimensional, and we are looking for a curve to approximate how to calculate one variable from another.
We will come across the following types of regression in this chapter:
- Linear regression with one variable using a polynomial of degree 1: This is the most basic form of regression, where a straight line approximates the trajectory of future data.
- Linear regression with multiple variables using a polynomial of degree 1: We will be using equations of degree 1, but we will also allow multiple input variables, called features.
- Polynomial regression with one variable: This is a generic form of the linear regression of one variable. As the polynomial used to approximate the relationship between the input and the output is of an arbitrary degree, we can create curves that fit the data points better than a straight line. The regression is still linear – not because the polynomial is linear, but because the regression problem can be modeled using linear algebra.
- Polynomial regression with multiple variables: This is the most generic regression problem, using higher degree polynomials and multiple features to predict the future.
- SVR: This form of regression uses Support Vector Machines (SVMs) to predict data points. This type of regression is included to explain SVR's usage compared to the other four regression types.
Now we will deal with the first type of linear regression: we will use one variable, and the polynomial of the regression will describe a straight line.
On the two-dimensional plane, we will use the Déscartes coordinate system, more commonly known as the Cartesian coordinate system. We have an x and a y-axis, and the intersection of these two axes is the origin. We denote points by their x and y coordinates.
For instance, point (2, 1) corresponds to the black point on the following coordinate system:

Figure 2.1: Representation of point (2,1) on the coordinate system
A straight line can be described with the equation y = a*x + b, where a is the slope of the equation, determining how steeply the equation climbs up, and b is a constant determining where the line intersects the y-axis.
In Figure 2.2, you can see three equations:
- The straight line is described with the equation y = 2*x + 1.
- The dashed line is described with the equation y = x + 1.
- The dotted line is described with the equation y = 0.5*x + 1.
You can see that all three equations intersect the y-axis at 1, and their slope is determined by the factor by which we multiply x.
If you know x, you can solve y. Similarly, if you know y, you can solve x. This equation is a polynomial equation of degree 1, which is the base of linear regression with one variable:

Figure 2.2: Representation of the equations y = 2*x + 1, y = x + 1, and y = 0.5*x + 1 on the coordinate system
We can describe curves instead of straight lines using polynomial equations; for example, the polynomial equation 4x4-3x3-x2-3x+3 will result in Figure 2.3. This type of equation is the base of polynomial regression with one variable:

Figure 2.3: Representation of the polynomial equation
Note
If you would like to experiment further with the Cartesian coordinate system, you can use the following plotter: https://s3-us-west-2.amazonaws.com/oerfiles/College+Algebra/calculator.html.
Features and Labels
In machine learning, we differentiate between features and labels. Features are considered our input variables, and labels are our output variables.
When talking about regression, the possible value of the labels is a continuous set of rational numbers. Think of features as the values on the x-axis and labels as the values on the y-axis.
The task of regression is to predict label values based on feature values.
We often create a label by projecting the values of a feature in the future.
For instance, if we would like to predict the price of a stock for next month using historical monthly data, we would create the label by shifting the stock price feature one month into the future:
- For each stock price feature, the label would be the stock price feature of the next month.
- For the last month, prediction data would not be available, so these values are all NaN (Not a Number).
Let's say we have data for the months of January, February, and March, and we want to predict the price for April. Our feature for each month will be the current monthly price and the label will be the price of the next month.
For instance, take a look at the following table:

Figure 2.4: Example of a feature and a label
This means that the label for January is the price of February and that the label for February is actually the price of March. The label for March is unknown (NaN) as this is the value we are trying to predict.
Feature Scaling
At times, we have multiple features (inputs) that may have values within completely different ranges. Imagine comparing micrometers on a map to kilometers in the real world. They won't be easy to handle because of the difference in magnitude of nine zeros.
A less dramatic difference is the difference between imperial and metric data. For instance, pounds and kilograms, and centimeters and inches, do not compare that well.
Therefore, we often scale our features to normalized values that are easier to handle, as we can compare the values of these ranges more easily.
We will demonstrate two types of scaling:
- Min-max normalization
- Mean normalization
Min-max normalization is calculated as follows:

Here, XMIN is the minimum value of the feature and XMAX is the maximum value.
The feature-scaled values will be within the range of [0;1].
Mean normalization is calculated as follows:

Here, AVG is the average.
The feature-scaled values will be within the range of [-1;1].
Here's an example of both normalizations applied on the first 13 numbers of the Fibonacci sequence.
We begin with finding the min-max normalization:
fibonacci = [0, 1, 1, 2, 3, 5, 8, 13, 21, 34, 55, 89, 144]
# Min-Max normalization:
[(float(i)-min(fibonacci))/(max(fibonacci)-min(fibonacci)) \
for i in fibonacci]
The expected output is this:
[0.0,
0.006944444444444444,
0.006944444444444444,
0.013888888888888888,
0.020833333333333332,
0.034722222222222224,
0.05555555555555555,
0.09027777777777778,
0.14583333333333334,
0.2361111111111111,
0.3819444444444444,
0.6180555555555556,
1.0]
Now, take a look at the following code snippet to find the mean normalization:
# Mean normalization:
avg = sum(fibonacci) / len(fibonacci)
# 28.923076923076923
[(float(i)-avg)/(max(fibonacci)-min(fibonacci)) \
for i in fibonacci]
The expected output is this:
[-0.20085470085470086,
-0.19391025641025642,
-0.19391025641025642,
-0.18696581196581197,
-0.18002136752136752,
-0.16613247863247863,
-0.1452991452991453,
-0.11057692307692307,
-0.05502136752136752,
0.035256410256410256,
0.18108974358974358,
0.4172008547008547,
0.7991452991452992]
Note
Scaling could add to the processing time, but, often, it is an important step to add.
In the scikit-learn library, we have access to the preprocessing.scale function, which scales NumPy arrays:
import numpy as np
from sklearn import preprocessing
preprocessing.scale(fibonacci)
The expected output is this:
array([-0.6925069 , -0.66856384, -0.66856384, -0.64462079,
-0.62067773-0.57279161, -0.50096244, -0.38124715,
-0.18970269, 0.12155706, 0.62436127, 1.43842524,
2.75529341]
The scale method performs a standardization, which is another type of normalization. Notice that the result is a NumPy array.
Splitting Data into Training and Testing
Now that we have learned how to normalize our dataset, we need to learn about the training-testing split. In order to measure how well our model can generalize its predictive performance, we need to split our dataset into a training set and a testing set. The training set is used by the model to learn from so that it can build predictions. Then, the model will use the testing set to evaluate the performance of its prediction.
When we split the dataset, we first need to shuffle it to ensure that our testing set will be a generic representation of our dataset. The split is usually 90% for the training set and 10% for the testing set.
With training and testing, we can measure whether our model is overfitting or underfitting.
Overfitting occurs when the trained model fits the training dataset too well. The model will be very accurate on the training data, but it will not be usable in real life, as its accuracy will decrease when used on any other data. The model adjusts to the random noise in the training data and assumes patterns on this noise that yield false predictions.
Underfitting occurs when the trained model does not fit the training data well enough to recognize important patterns in the data. As a result, it cannot make accurate predictions on new data. One example of this is when we attempt to do linear regression on a dataset that is not linear. For example, the Fibonacci sequence is not linear; therefore, a model on a Fibonacci-like sequence cannot be linear either.
We can do the training-testing split using the model_selection library of scikit- learn.
Suppose, in our example, that we have scaled the Fibonacci data and defined its indices as labels:
features = preprocessing.scale(fibonacci)
label = np.array(range(13))
Now, let's use 10% of the data as test data, test_size=0.1, and specify random_state parameter in order to get the exact same split every time we run the code:
from sklearn import model_selection
(x_train, x_test, y_train, y_test) = \
model_selection.train_test_split(features, \
label, test_size=0.1, \
random_state=8)
Our dataset has been split into test and training sets for our features (x_train and x_test) and for our labels (y_train and y_test).
Finally, let's check each set, beginning with the x_train feature:
x_train
The expected output is this:
array([ 1.43842524, -0.18970269, -0.50096244, 2.75529341,
-0.6925069 , -0.66856384, -0.57279161, 0.12155706,
-0.66856384, -0.62067773, -0.64462079])
Next, we check for x_test:
x_test
The expected output is this:
array([-0.38124715, 0.62436127])
Then, we check for y_train:
y_train
The expected output is this:
array([11, 8, 6, 12, 0, 2, 5, 9, 1, 4, 3])
Next, we check for y_test:
y_test
The expected output is this:
array([7, 10])
In the preceding output, we can see that our split has been properly executed; for instance, our label has been split into y_test, which contains the 7 and 10 indexes, and y_train which contains the remaining 11 indexes. The same logic has been applied to our features and we have 2 values in x_test and 11 values in x_train.
Note
If you remember the Cartesian coordinate system, you know that the horizontal axis is the x-axis and that the vertical axis is the y-axis. Our features are on the x-axis, while our labels are on the y-axis. Therefore, we use features and x as synonyms, while labels are often denoted by y. Therefore, x_test denotes feature test data, x_train denotes feature training data, y_test denotes label test data, and y_train denotes label training data.
Fitting a Model on Data with scikit-learn
We are now going to illustrate the process of regression on an example where we only have one feature and minimal data.
As we only have one feature, we have to format x_train by reshaping it with x_train.reshape (-1,1) to a NumPy array containing one feature.
Therefore, before executing the code on fitting the best line, execute the following code:
x_train = x_train.reshape(-1, 1)
x_test = x_test.reshape(-1, 1)
We can fit a linear regression model on our data with the following code:
from sklearn import linear_model
linear_regression = linear_model.LinearRegression()
model = linear_regression.fit(x_train, y_train)
model.predict(x_test)
The expected output is this:
array([4.46396931, 7.49212796])
We can also calculate the score associated with the model:
model.score(x_test, y_test)
The expected output is this:
-1.8268608450379087
This score represents the accuracy of the model and is defined as the R2 or coefficient of determination. It represents how well we can predict the features from the labels.
In our example, an R2 of -1.8268 indicates a very bad model as the best possible score is 1. A score of 0 can be achieved if we constantly predict the labels by using the average value of the features.
Note
We will omit the mathematical background of this score in this book.
Our model does not perform well for two reasons:
- If we check our previous Fibonacci sequence, 11 training data points and 2 testing data points are simply not enough to perform a proper predictive analysis.
- Even if we ignore the number of points, the Fibonacci sequence does not describe a linear relationship between x and y. Approximating a nonlinear function with a line is only useful if we are looking at two very close data points.
Linear Regression Using NumPy Arrays
One reason why NumPy arrays are handier than Python lists is that they can be treated as vectors. There are a few operations defined on vectors that can simplify our calculations. We can perform operations on vectors of similar lengths.
Let's take, for example, two vectors, V1 and V2, with three coordinates each:
V1 = (a, b, c) with a=1, b=2, and c=3
V2 = (d, e, f) with d=2, e=0, and f=2
The addition of these two vectors will be this:
V1 + V2 = (a+d, b+e, c+f) = (1+2, 2+0, 3+2) = (3,2,5)
The product of these two vectors will be this:
V1 + V2 = (a*d, b*e, c*f) = (1*2, 2*0, 3*2) = (2,0,6)
You can think of each vector as our datasets with, for example, the first vector as our features set and the second vector as our labels set. With Python being able to do vector calculations, this will greatly simplify the calculations required for our linear regression models.
Now, let's build a linear regression using NumPy in the following example.
Suppose we have two sets of data with 13 data points each; we want to build a linear regression that best fits all the data points for each set.
Our first set is defined as follows:
[2, 8, 8, 18, 25, 21, 32, 44, 32, 48, 61, 45, 62]
If we plot this dataset with the values (2,8,8,18,25,21,32,44,32,48,61,45,62) as the y-axis, and the index of each value (1,2,3,4,5,6,7,8,9,10,11,12,13) as the x-axis, we will get the following plot:

Figure 2.5: Plotted graph of the first dataset
We can see that this dataset's distribution seems linear in nature, and if we wanted to draw a line that was as close as possible to each dot, it wouldn't be too hard. A simple linear regression appears appropriate in this case.
Our second set is the first 13 values scaled in the Fibonacci sequence that we saw earlier in the Feature Scaling section:
[-0.6925069, -0.66856384, -0.66856384, -0.64462079, -0.62067773, -0.57279161, -0.50096244, -0.38124715, -0.18970269, 0.12155706, 0.62436127, 1.43842524, 2.75529341]
If we plot this dataset with the values as the y-axis and the index of each value as the x-axis, we will get the following plot:

Figure 2.6: Plotted graph of the second dataset
We can see that this dataset's distribution doesn't appear to be linear, and if we wanted to draw a line that was as close as possible to each dot, our line would miss quite a lot of dots. A simple linear regression will probably struggle in this situation.
We know that the equation of a straight line is .
In this equation, is the slope, and
is the y intercept. To find the line of best fit, we must find the coefficients of
and
.
In order to do this, we will use the least-squares method, which can be achieved by completing the following steps:
- For each data point, calculate x2 and xy.
Sum all of x, y, x2, and x * y, which gives us
- Calculate the slope,
, as
with N as the total number of data points.
- Calculate the y intercept,
, as
.
Now, let's apply these steps using NumPy as an example for the first dataset in the following code.
Let's take a look at the first step:
import numpy as np
x = np.array(range(1, 14))
y = np.array([2, 8, 8, 18, 25, 21, 32, 44, 32, 48, 61, 45, 62])
x_2 = x**2
xy = x*y
For x_2, the output will be this:
array([ 1, 4, 9, 16, 25, 36, 49, 64, 81,
100, 121, 144, 169], dtype=int32)
For xy, the output will be this:
array([2, 16, 24, 72, 125, 126, 224,
352, 288, 480, 671, 540, 806])
Now, let's move on to the next step:
sum_x = sum(x)
sum_y = sum(y)
sum_x_2 = sum(x_2)
sum_xy = sum(xy)
For sum_x, the output will be this:
91
For sum_y, the output will be this:
406
For sum_x_2, the output will be this:
819
For sum_xy, the output will be this:
3726
Now, let's move on to the next step:
N = len(x)
a = (N*sum_xy - (sum_x*sum_y))/(N*sum_x_2-(sum_x)**2)
For N, the output will be this:
13
For a, the output will be this:
4.857142857142857
Now, let's move on to the final step:
b = (sum_y - a*sum_x)/N
For b, the output will be this:
-2.7692307692307647
Once we plot the line with the preceding coefficients, we get the following graph:

Figure 2.7: Plotted graph of the linear regression for the first dataset
As you can see, our linear regression model works quite well on this dataset, which has a linear distribution.
Note
You can find a linear regression calculator at http://www.endmemo.com/statistics/lr.php. You can also check the calculator to get an idea of what lines of best fit look like on a given dataset.
We will now repeat the exact same steps for the second dataset:
import numpy as np
x = np.array(range(1, 14))
y = np.array([-0.6925069, -0.66856384, -0.66856384, \
-0.64462079, -0.62067773, -0.57279161, \
-0.50096244, -0.38124715, -0.18970269, \
0.12155706, 0.62436127, 1.43842524, 2.75529341])
x_2 = x**2
xy = x*y
sum_x = sum(x)
sum_y = sum(y)
sum_x_2 = sum(x_2)
sum_xy = sum(xy)
N = len(x)
a = (N*sum_xy - (sum_x*sum_y))/(N*sum_x_2-(sum_x)**2)
b = (sum_y - a*sum_x)/N
For a, the output will be this:
0.21838173510989017
For b, the output will be this:
-1.528672146538462
Once we plot the line with the preceding coefficients, we get the following graph:

Figure 2.8: Plotted graph of the linear regression for the second dataset
Clearly, with a nonlinear distribution, our linear regression model struggles to fit the data.
Note
We don't have to use this method to perform linear regression. Many libraries, including scikit-learn, will help us to automate this process. Once we perform linear regression with multiple variables, we are better off using a library to perform the regression for us.
Fitting a Model Using NumPy Polyfit
NumPy Polyfit can also be used to create a line of best fit for linear regression with one variable.
Recall the calculation for the line of best fit:
import numpy as np
x = np.array(range(1, 14))
y = np.array([2, 8, 8, 18, 25, 21, 32, 44, 32, 48, 61, 45, 62])
x_2 = x**2
xy = x*y
sum_x = sum(x)
sum_y = sum(y)
sum_x_2 = sum(x_2)
sum_xy = sum(xy)
N = len(x)
a = (N*sum_xy - (sum_x*sum_y))/(N*sum_x_2-(sum_x)**2)
b = (sum_y - a*sum_x)/N
The equation for finding the coefficients and
is quite long. Fortunately, numpy.polyfit in Python performs these calculations to find the coefficients of the line of best fit. The polyfit function accepts three arguments: the array of x values, the array of y values, and the degree of polynomial to look for. As we are looking for a straight line, the highest power of x is 1 in the polynomial:
import numpy as np
x = np.array(range(1, 14))
y = np.array([2, 8, 8, 18, 25, 21, 32, 44, 32, 48, 61, 45, 62])
[a,b] = np.polyfit(x, y, 1)
For [a,b], the output will be this:
[4.857142857142858, -2.769230769230769]
Plotting the Results in Python
Suppose you have a set of data points and a regression line; our task is to plot the points and the line together so that we can see the results with our eyes.
We will use the matplotlib.pyplot library for this. This library has two important functions:
- scatter: This displays scattered points on the plane, defined by a list of x coordinates and a list of y coordinates.
- plot: Along with two arguments, this function plots a segment defined by two points or a sequence of segments defined by multiple points. A plot is like a scatter, except that instead of displaying the points, they are connected by lines.
A plot with three arguments plots a segment and/or two points formatted according to the third argument.
A segment is defined by two points. As x ranges between 1 and 13 (remember the dataset contains 13 data points), it makes sense to display a segment between 0 and 15. We must substitute the value of x in the equation to get the corresponding y values:
import numpy as np
import matplotlib.pyplot as plot
x = np.array(range(1, 14))
y = np.array([2, 8, 8, 18, 25, 21, 32, 44, 32, 48, 61, 45, 62])
x_2 = x**2
xy = x*y
sum_x = sum(x)
sum_y = sum(y)
sum_x_2 = sum(x_2)
sum_xy = sum(xy)
N = len(x)
a = (N*sum_xy - (sum_x*sum_y))/(N*sum_x_2-(sum_x)**2)
b = (sum_y - a*sum_x)/N
# Plotting the points
plot.scatter(x, y)
# Plotting the line
plot.plot([0, 15], [b, 15*a+b])
plot.show()
The output is as follows:

Figure 2.9: Plotted graph of the linear regression for the first dataset using matplotlib
The regression line and the scattered data points are displayed as expected.
However, the plot has an advanced signature. You can use plot to draw scattered dots, lines, and any curves on this figure. These variables are interpreted in groups of three:
- x values
- y values
- Formatting options in the form of a string
Let's create a function for deriving an array of approximated y values from an array of approximated x values:
def fitY( arr ):
return [4.857142857142859 * x - 2.7692307692307843 for x in arr]
We will use the fit function to plot the values:
plot.plot(x, y, 'go',x, fitY(x), 'r--o')
Every third argument handles formatting. The letter g stands for green, while the letter r stands for red. You could have used b for blue and y for yellow, among other examples. In the absence of a color, each triple value will be displayed using a different color. The o character symbolizes that we want to display a dot where each data point lies. Therefore, go has nothing to do with movement – it requests the plotter to plot green dots. The - characters are responsible for displaying a dashed line. If you just use -1, a straight line appears instead of the dashed line.
The output is as follows:

Figure 2.10: Graph for the plot function using the fit function
The Python plotter library offers a simple solution for most of your graphing problems. You can draw as many lines, dots, and curves as you want on this graph.
When displaying curves, the plotter connects the dots with segments. Also, bear in mind that even a complex sequence of curves is an approximation that connects the dots. For instance, if you execute the code from https://gist.github.com/traeblain/1487795, you will recognize the segments of the batman function as connected lines:

Figure 2.11: Graph for the batman function
There is a large variety of ways to plot curves. We have seen that the polyfit method of the NumPy library returns an array of coefficients to describe a linear equation:
import numpy as np
x = np.array(range(1, 14))
y = np.array([2, 8, 8, 18, 25, 21, 32, 44, 32, 48, 61, 45, 62])
np.polyfit(x, y, 1)
Here the output is as follows:
[4.857142857142857, -2.769230769230768]
This array describes the equation 4.85714286 * x - 2.76923077.
Suppose we now want to plot a curve, . This quadratic equation is described by the coefficient array [-1, 3, -2] as
. We could write our own function to calculate the y values belonging to x values. However, the NumPy library already has a feature that can do this work for us – np.poly1d:
import numpy as np
x = np.array(range( -10, 10, 1 ))
f = np.poly1d([-1,3,-2])
The f function that's created by the poly1d call not only works with single values but also with lists or NumPy arrays:
f(5)
The expected output is this:
-12
Similarly, for f(x):
f(x)
The output will be:
array ([-132. -110, -90, -72, -56, -42, -30, -20, -12, -6, -2,
0, 0, -2, -6, -12, -20, -30, -42, -56])
We can now use these values to plot a nonlinear curve:
import matplotlib.pyplot as plot
plot.plot(x, f(x))
The output is as follows:

Figure 2.12: Graph for a nonlinear curve
As you can see, we can use the pyplot library to easily create the plot of a nonlinear curve.
Predicting Values with Linear Regression
Suppose we are interested in the y value belonging to the x coordinate 20. Based on the linear regression model, all we need to do is substitute the value of 20 in the place of x on the previously used code:
x = np.array(range(1, 14))
y = np.array([2, 8, 8, 18, 25, 21, 32, 44, 32, 48, 61, 45, 62])
# Plotting the points
plot.scatter(x, y)
# Plotting the prediction belonging to x = 20
plot.scatter(20, a * 20 + b, color='red')
# Plotting the line
plot.plot([0, 25], [b, 25*a+b])
The output is as follows:

Figure 2.13: Graph showing the predicted value using linear regression
Here, we denoted the predicted value with red. This red point is on the best line of fit.
Let's look at next exercise where we will be predicting populations based on linear regression.
Exercise 2.01: Predicting the Student Capacity of an Elementary School
In this exercise, you will be trying to forecast the need for elementary school capacity. Your task is to figure out 2025 and 2030 predictions for the number of children starting elementary school.
Note
The data is contained inside the population.csv file, which you can find on our GitHub repository: https://packt.live/2YYlPoj.
The following steps will help you to complete this exercise:
- Open a new Jupyter Notebook file.
- Import pandas and numpy:
import pandas as pd
import numpy as np
import matplotlib.pyplot as plot
- Next, load the CSV file as a DataFrame on the Notebook and read the CSV file:
Note
Watch out for the slashes in the string below. Remember that the backslashes ( \ ) are used to split the code across multiple lines, while the forward slashes ( / ) are part of the URL.
file_url = 'https://raw.githubusercontent.com/'\
'PacktWorkshops/The-Applied-Artificial-'\
'Intelligence-Workshop/master/Datasets/'\
'population.csv'
df = pd.read_csv(file_url)
df
The expected output is this:
Figure 2.14: Reading the CSV file
- Now, convert the DataFrame into two NumPy arrays. For simplicity, we can indicate that the year feature, which is from 2001 to 2018, is the same as 1 to 18:
x = np.array(range(1, 19))
y = np.array(df['population'])
The x output will be:
array([1, 2, 3, 4, 5, 6, 7, 8, 9, 10, 11, 12, 13, 14, 15, 16, 17, 18])
The y output will be:
array([147026, 144272, 140020, 143801, 146233,
144539, 141273, 135389, 142500, 139452,
139722, 135300, 137289, 136511, 132884,
125683, 127255, 124275], dtype=int64)
- Now, with the two NumPy arrays, use the polyfit method (with a degree of 1 as we only have one feature) to determine the coefficients of the regression line:
[a, b] = np.polyfit(x, y, 1)
The output for [a, b] will be:
[-1142.0557275541803, 148817.5294117647]
- Now, plot the results using matplotlib.pyplot and predict the future until 2030:
plot.scatter( x, y )
plot.plot( [0, 30], [b, 30*a+b] )
plot.show()
The expected output is this:
Figure 2.15: Plot showing the future for 2030
As you can see, the data appears linear and our model seems to be a good fit.
- Finally, predict the population for 2025 and 2030:
population_2025 = 25*a+b
population_2030 = 30*a+b
The output for population_2025 will be:
120266.1362229102
The output for population_2030 will be:
114555.85758513928
Note
To access the source code for this specific section, please refer to https://packt.live/31dvuKt.
You can also run this example online at https://packt.live/317qeIc. You must execute the entire Notebook in order to get the desired result.
By completing this exercise, we can now conclude that the population of children starting elementary school is going to decrease in the future and that there is no need to increase the elementary school capacity if we are currently meeting the needs.