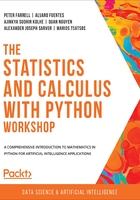
Introduction
So far, we have learned how to use the Python language, especially three of its core libraries—NumPy, pandas, and Matplotlib, for statistics and data science. However, in order to fully take advantage of these tools, we will need to have a solid theoretical understanding of statistics itself. By knowing the idea behind statistical tests and techniques, we will be able to utilize the tools that Python offers more effectively.
It is true that in statistics and machine learning, libraries in Python offer great options—from data cleaning/processing to modeling and making inferences. However, a fundamental understanding of statistics is still required so that we can make initial decisions regarding what kinds of techniques should be used in our process, depending on the data we have.
As such, in this chapter, we will learn about core concepts in statistics such as , inference, sampling, variables, and so on. We will also be introduced to a wide range of Python tools that can help facilitate more advanced statistical techniques and needs. All of this will be demonstrated with hands-on discussions and examples.