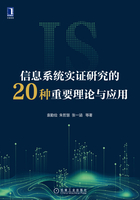
3.2.1 以确认后阶段为核心的适应性研究
作为确认阶段的起点,确认水平的形成过程首先会受到研究者的关注,包括其度量方式及其可能受到的影响等。其次,在应用模型时为了应对研究对象和研究场景的差异性,多维度的事后期望构建成为大多数学者的选择。虽然对确认过程和事后期望的探讨丰富了原有的研究视角,也从多角度探究了影响用户态度的因素,但若想获得真正的实践收益,停留在感知态度层的研究成果的说服力就略显不足。因此,对用户意愿向行为转化过程的了解便显得尤为重要。故确认后阶段的研究评述将从以上三个方面展开。
1. 确认过程的影响
在期望确认理论的相关研究中,主要有三种度量确认水平的方式:客观型(Objective)、推断型(Inferred)和感知型(Perceived)[1]。客观度量需要一名外部裁判“客观地”衡量期望与实际绩效间的差异,但是这一判断有一个假设前提,即基于事前定义的明晰标准,不同的消费者会对产品或服务有一致的评价[2]。显然,这一前提条件忽略了消费者对绩效表现和期望确认度感知时的个体差异。因此,尽管客观度量易于操作,但其对满意度的前瞻性预测还不够准确。[3]而推断度量是就预先设定的产品或服务属性,计算期望与感知绩效间的差异,其评估的是消费者在消费体验前后,评价预选属性的差异分数的总和[4],也常被表示为期望与感知绩效间代数差的函数[5]。感知度量得到的确认水平则是消费者事后对期望与感知绩效间差异的主观评价,这一主观评价或基于产品和服务的整体水平[6],或基于个人的属性水平[7]。在针对信息系统的研究中,往往不存在明晰的评价标准,学者们也常以探究用户的期望点为目标,所以客观型度量并不适用。同时,尽管推断型度量在衡量具有明确侧重属性的期望与绩效差异时较为适用[8],但在分别测度时,绩效因为是后置测量而可能会受到期望的影响,实际水平可能不够准确[9]。于是,感知型测度自然成为学者们最常采用的度量确认水平的方式。
虽然在原理论模型中,确认水平同时受到期望和感知绩效的影响,但是在采用感知度量的方式后,研究者常以确认水平代表事前期望和感知绩效的综合影响,不去理会其中更细致的影响关系,转而关注其他可能影响确认水平的因素。首先,从客观条件的支持来看,较好的服务质量可以为用户确认期望提供有力支持从而得到积极的确认结果[10];进一步而言,基于良好的服务质量或环境支持带来的用户专注度的提升也可以促进期望确认水平。其次,从用户的主观条件出发,以往的经验与习惯成为主要的考察对象[11][12][13][14][15],以往积极的使用体验会为当下的确认过程奠定良好的基础;而且基于自我觉知理论,用户如果经常使用某一信息系统或产品,他会认为这是出于喜欢的行为,从而在评价期望确认程度时也是积极的。此外,频繁的使用行为也可以理解为形成了一种依赖关系[16],加深了用户的感知印象,促进了积极确认结果的形成。同时,用户也会受到他人行为或评价的影响,从而改变自身对确认水平的判断。[17]
由上可知,感知型的度量方式因为融合了事前期望和感知绩效的影响,减少了对确认水平形成过程的关注。确认水平在模型中的位置前移,也使得现有的大部分研究将重心置于吸纳了确认水平影响的事后期望。然而,若想细致了解完整的期望确认过程,确认阶段作为承接环节的作用也是不应忽视的。同时,确认过程对事后期望的影响是否会受到诸如用户特征、环境特征等因素的调节也缺少进一步的探讨,需要在整体的研究框架中填补更多的细节。
2. 事后期望的构建
当事前期望由于难以捕捉被IS持续使用模型舍弃时,事后期望的构建可视为对事前期望的一种探索,并作为联结确认水平与满意度水平之间的桥梁。学者们根据研究对象的特点具体划分事后期望的维度,勾勒出影响用户满意度的路径,以给出更加具有针对性、实践意义和操作价值的建议,进而促进用户忠诚度和持续使用意愿的形成。
虽然称之为事后期望,在实际研究测度时却倾向于考察用户对实际体验的感知情况(以感知有用性为例,该构念的实际测量项旨在衡量使用该系统是否提升了个人处理相关事务时的绩效),所以事后期望实则是融合了期望与感知绩效对实际体验的评估和更进一步的确认,也可以理解为指向性更为明确的期望再确认,或是衡量用户对信息系统产品不同方面价值的感知水平[18]。
已有研究中提出的事后期望主要有两方面来源—理论支撑和情境支撑。
由于在期望确认理论中,期望仅作为一个宽泛的概念,其具体的内涵需要学者根据研究的对象和目的进行细化,而现有的较为成熟的理论,则为学者们探究期望的维度提供了丰富的理论支持。因为IS持续使用模型自身即借鉴了技术接受模型,所以其中没有囊括的感知易用性的影响成为后续研究的一个关注点。尽管有研究指出,感知易用性的作用会随着用户对系统熟悉度的增加而减少,因而在技术采纳后的阶段不会存在显著影响,但Hong等的研究指出,感知易用性对于满意度和持续使用意愿都有积极影响,甚至强于感知有用性的影响,尤其是对功能和内容处于不断扩展过程中的移动网络而言,用户在使用设备时与传统渠道相比需要付出更多努力。[19]而用户使用信息系统时的限制条件也可以通过用户对完成某一项行为所需资源或机会的可及性感知来测度,即计划行为理论中的感知行为控制。同时,该理论提出的主观规范的影响也被引入对IS持续使用意愿的研究中[20]。Hsu等的研究表明,社会关系的影响和感知行为控制都会受到确认水平的积极影响,从而促进持续使用意愿的形成。[21]结合了技术接受模型,计划行为理论的研究都将IS的持续使用作为单向因果关系来考虑,认为环境影响认知信念,进而影响态度和行为。而社会认知理论则给出了另一种模式,提出环境因素、认知因素和人类行为之间存在持续的相互因果关系。[22]
事后期望的维度也代表着用户使用信息系统的不同动机。借助自我决定理论对动机的划分,事后期望可细化为促进满意度提升和持续使用意愿形成的内在动机(偏向享乐性)与外在动机(偏向有用性),以及支撑动机的基础心理需求。研究结果表明,迎合用户的心理动机带来的积极影响要明显强于满意度对持续使用意愿的作用。[23]为了多角度探究用户的需要,Yeh等结合了生存、相互关系、成长发展三核心需要理论(Existence Relatedness Growth Theory,ERG),在扩展有用性内涵的同时,也关注了人们对相互关系(Relatedness)的需要和成长发展(Growth)的需要,证实了感知需求的满足对持续使用意愿的积极影响。[24]此外,整合型技术接受模型也是一个常见的选择,它包含绩效期望、付出期望、社群影响和配合情况四个核心维度。前两者与期望确认模型中的期望相契合,同时,纳入的社会因素和环境的支持条件在信息系统的使用情境中也是十分重要的影响因素。这四个维度的期望在受到确认水平的影响后也会改变用户的态度。而态度相对于满意度而言,是一个长期且稳定的心理认知,其对后续使用意愿的影响也得到了证实。[25]
虽然从理论中提取的期望维度界定清晰、指向明确,但在实证研究中也需要根据研究对象或使用情境予以调整。例如,线下环境中易用性的作用可能更突出,而线上环境中用户更加关注辅助条件提供的帮助是否充足。[26]所以结合使用情境理解影响用户认知和态度的变化是非常重要的,这也促成了事后期望构建的另一个主要来源。
已有文献所研究的信息系统对象主要有以下四种类型:技术型、服务型、社交型和娱乐型。在构建期望时,技术型侧重于衡量有用性与易用性,服务型强调感知价值与收益,社交型偏向于情感维系,而娱乐型注重享乐体验。这些属性并非彼此独立,它们往往糅合于同一信息系统工具中,以满足用户多层次的需求。从更宏观的角度来说,在根据研究对象的应用情境构建期望时,可将之分为绩效型期望和情感型期望两方面。绩效型期望主要衡量的是用户在实际体验中预期获得的价值,也考虑了为此付出的努力或代价。这种价值可以用净收益(即感知价值)表示[27],也可以由感知收益和感知成本两方面分别测度[28]。感知成本包括使用信息系统或相关产品和服务需要付出的金钱成本[29]、学习成本[30]、习惯转变成本[31]等。感知成本可视为用户使用信息系统的阻碍,会对用户满意度产生消极影响,但较高的期望确认水平则会降低用户对于成本的感知。[32]此外,辅助条件的支持也会降低感知成本,从而增强用户的使用意愿[33]。感知收益的衡量除了相对宽泛的感知有用性评估外,还包括对服务质量[34][35]、金钱价值、绩效收益[36]等方面的评估。感知收益是用户采纳信息系统的动因,与用户的满意度正相关,在用户感知信息系统或产品有用的前提下,辅以增强用户黏性的措施,将会进一步增强用户的持续使用意愿。[37]
绩效型期望对技术型、服务型的IS工具而言是一种重要的度量项。而考察用户心理状态和情绪体验的情感型期望,则会对娱乐型、社交型的IS工具形成更加显著和直接的影响。感知娱乐性(Perceived Playfulness)和感知享乐性(Perceived Enjoyment)是常见的情感型期望构念,旨在衡量用户在使用信息系统的过程中所获得的愉悦感以及用户是否享受于整个使用过程。它们对具有社交属性的产品(如社区[38]、博客[39])和娱乐属性的产品(如门户网站[40]、游戏[41])的持续使用意愿都存在积极影响。除了短期使用获得的娱乐享受外,用户对信息系统产品的情感依赖还可以体现为长期使用形成的熟悉度和亲密度。针对在线购物网站的研究表明,这二者同样会受到确认水平的影响,并直接影响用户的持续使用意愿,其作用甚至强于感知有用性[42]。此外,由于用户在使用新兴技术传输个人信息和敏感信息时越来越多地注重隐私安全问题,信任因素也被纳入了相关情境的研究中[43][44][45],尤其是对隐私信息敏感度较高的移动医疗服务而言,感知信任度会显著影响持续使用意愿[46]。
如前所述,基于理论融合和情境化视角构建的事后期望使得模型可以针对不同的研究对象调整侧重点,积累了较为丰富的研究成果。但是,现有研究从其他理论抽取的构念往往过于笼统,没有进行较细维度的划分(以感知有用性为例,研究者多以整体有用性为出发点,缺少对具体功能板块有用性的考察)。同时,这一方法对不同类型的期望间的影响关系也缺乏进一步探讨,忽略了其中可能存在的单向影响或交互作用(例如,绩效型期望的满足是否会对情感型期望的确认具有促进作用)。未来的研究可以考虑做此方面的补充,以提供更具实践价值的建议。
3. 用户意愿的转化
基于IS持续使用模型的研究多将持续使用意愿作为预测持续使用行为的主要因素,虽然这一点没有在模型中明确指出,但研究者在分析结果时往往将意愿与行为联系在一起。然而,由于不包含行为构念,模型不能够解释长期行为可能被其他因素(如习惯)影响而不受意愿控制的状况。
为了克服这些局限性,进一步提高模型的解释力,研究者们探讨了可能影响用户意愿向行为转化的因素。有别于“有意识”的意愿,习惯被视为一种“无意识”因素。习惯基于过往行为形成,并受到使用环境、使用体验的丰富性和满意度的影响。[47]它会调节意愿与持续使用行为间的关系,即习惯性越强,意愿对实际行为的影响越小。而意愿在相对不稳定的或变化较大的环境中对后续行为的影响将会更强。[48]除了习惯外,如果行为的实施过程有便利条件的支持,也会促进意愿向行为的转化。[49][50]
总体来看,作为最终实现研究价值的重要环节,意愿向行为的转化过程却没有受到应有的关注。造成这一现状的原因首先是受期望确认理论模型和IS持续使用模型的惯性思维影响,以意愿的形成作为模型的最终目标;其次是由于行为数据不能基于问卷获得,这对研究者的研究设计和数据获取提出了更高的要求。未来的研究可以考虑纳入行为数据进行分析,或可将行为的实现视为对意愿的一种确认,并将影响确认过程的因素纳入考量,以作为对意愿性研究成果的补充,提供更全面的研究结论。
[1] Yi Y. A Critical Review of Consumer Satisfaction[M]// Zeithaml V. A. Review of Marketing 1990. Chicago IL: American Marketing Association, 1991: 68-123.
[2] Olshavsky R W, Miller J A. Consumer expectations, product performance, and perceived product quality[J]. Journal of Marketing Research, 1972, 9(1): 19-21.
[3] Yi Y. A Critical review of consumer satisfaction[M]// Zeithaml V. A. Review of Marketing 1990. Chicago IL: American Marketing Association, 1991: 68-123.
[4] Swan J E, Trawick I F. Disconfirmation of expectations and satisfaction with a retail service[J]. Journal of Retailing, 1981, 57(3): 49-67.
[5] Tse D K, Wilton P C. Models of consumer satisfaction: An extension[J]. Journal of Marketing Research, 1988, 25(2): 204-212.
[6] Oliver R L. A cognitive model of the antecedents and consequences of satisfaction decisions[J]. Journal of Marketing Research, 1980, 17(4): 460-469.
[7] Tse D K, Wilton P C. Models of consumer satisfaction: An extension[J]. Journal of Marketing Research, 1988, 25(2): 204-212.
[8] Terzis V, Moridis C N, Economides A A. Continuance acceptance of computer based assessment through the integration of user’s expectations and perceptions[J]. Computers and Education, 2013, 62: 50-61.
[9] Michael J. Roszkowski, John S. Baky, David B. Jones. So which score on the LibQual+ ™ tells me if library users are satisfied?[J]. Library and Information Science Research, 2005, 27(4): 424-439.
[10] Oghuma A P, Libaque-Saenz C F, Wong S F, et al. An expectation-confirmation model of continuance intention to use mobile instant messaging[J]. Telematics and Informatics, 2016, 33(1): 34-47.
[11] Recker J. Continued use of process modeling grammars: The impact of individual difference factors[J]. European Journal of Information Systems, 2010, 19(1): 76-92.
[12] Hung M C, Chang I C, Hwang H G. Exploring academic teachers’ continuance toward the web-based learning system: The role of causal attributions[J]. Computers and Education, 2011, 57(2): 1530-1543.
[13] Kim B, Han I. The role of trust belief and its antecedents in a community-driven knowledge environment[J]. Journal of the Association for Information Science and Technology, 2010, 60(5): 1012-1026.
[14] Shin, D H, Biocca F, Choo H. Exploring the user experience of three-dimensional virtual learning environments[J]. Behaviour and Information Technology, 2013, 32(2): 203-214.
[15] Chen Y Y. Why do consumers go internet shopping again? Understanding the antecedents of repurchase intention[J]. Journal of Organizational Computing and Electronic Commerce, 2012, 22(1): 38-63.
[16] 常桂林,毕强,费陆陆.微信平台(公众号)用户持续使用意愿分析—基于期望确认模型与媒介系统依赖理论[J].图书馆学研究,2017,(22): 85-92.
[17] Chen Y Y. Why do consumers go internet shopping again? Understanding the antecedents of repurchase intention[J]. Journal of Organizational Computing and Electronic Commerce, 2012, 22(1): 38-63.
[18] 杨根福.MOOC用户持续使用行为影响因素研究[J].开放教育研究,2016,22(1): 100-111.
[19] Hong S J, Thong J Y L, Tam K Y. Understanding continued information technology usage behavior: A comparison of three models in the context of mobile internet[J]. Decision Support Systems, 2007, 42(3): 1819-1834.
[20] Kim B. An empirical investigation of mobile data service continuance: Incorporating the theory of planned behavior into the expectation–confirmation model[J]. Expert Systems with Applications, 2010, 37(10): 7033-7039.
[21] Hsu M H, Yen C H, Chiu C M, et al. A longitudinal investigation of continued online shopping behavior: An extension of the theory of planned behavior[J]. International Journal of Human-Computer Studies, 2006, 64(9): 889-904.
[22] Wood R, Bandura A. Social Cognitive Theory of Organizational Management[J]. Academy of Management Review, 1989, 14(3): 361-384.
[23] Øystein Sørebø, Halvari H, Gulli V F, et al. The role of self-determination theory in explaining teachers’ motivation to continue to use e-learning technology[J]. Computers and Education, 2009, 53(4): 1177-1187.
[24] Yeh K J, Teng T C. Extended conceptualisation of perceived usefulness: Empirical test in the context of information system use continuance[J]. Behaviour and Information Technology, 2012, 31(5): 525-540.
[25] Venkatesh V, Thong J Y L, Chan F K Y, et al. Extending the two-stage information systems continuance model: Incorporating UTAUT predictors and the role of context[J]. Information Systems Journal, 2011, 21(6): 527-555.
[26] Venkatesh V, Thong J Y L, Chan F K Y, et al. Extending the two-stage information systems continuance model: Incorporating UTAUT predictors and the role of context[J]. Information Systems Journal, 2011, 21(6): 527-555.
[27] 殷猛,李琪.基于价值感知的微博话题持续参与意愿研究[J].情报杂志,2017,36(8): 94-100.
[28] Lin T C, Wu S, Hsu S C, et al. The integration of value-based adoption and expectation–confirmation models: An example of IPTV continuance intention[J]. Decision Support Systems, 2012, 54(1): 63-75.
[29] Kim B. An empirical investigation of mobile data service continuance: Incorporating the theory of planned behavior into the expectation–confirmation model[J]. Expert Systems with Applications, 2010, 37(10): 7033-7039.
[30] Lin T C, Wu S, Hsu S C, et al. The integration of value-based adoption and expectation–confirmation models: An example of IPTV continuance intention[J]. Decision Support Systems, 2012, 54(1): 63-75.
[31] Chong Y L. Understanding mobile commerce continuance intentions: An empirical analysis of Chinese consumers[J]. Data Processor for Better Business Education, 2013, 53(4): 22-30.
[32] Kim B. An empirical investigation of mobile data service continuance: Incorporating the theory of planned behavior into the expectation–confirmation model[J]. Expert Systems with Applications, 2010, 37(10): 7033-7039.
[33] Lankton N K, Wilson E V, Mao E. Antecedents and determinants of information technology habit[J]. Information and Management, 2010, 47(5): 300-307.
[34] Hsu M H, Chang C M, Chuang L W. Understanding the determinants of online repeat purchase intention and moderating role of habit: The case of online group-buying in China Taiwan[J]. International Journal of Information Management, 2015, 35(1): 45-56.
[35] Chen I Y L. The factors influencing members’ continuance intentions in professional virtual communities — A longitudinal study[J]. Journal of Information Science, 2007, 33(4): 451-467.
[36] Hsu C L, Lin C C. What drives purchase intention for paid mobile Apps? An expectation confirmation model with perceived value[J]. Electronic Commerce Research and Applications, 2015, 14(1): 46-57.
[37] Bhattacherjee A. An empirical analysis of the antecedents of electronic commerce service continuance[J]. Decision Support Systems, 2001, 32(2): 201-214.
[38] Alraimi K M, Zo H, Ciganek A P. Understanding the MOOCs continuance: The role of openness and reputation[J]. Computers and Education, 2015, 80: 28-38.
[39] Luo M M. Continuance intention of blog users: The impact of perceived enjoyment, habit, user involvement and blogging time[J]. Behaviour and Information Technology, 2013, 32(6): 570-583.
[40] Lin C S, Wu S, Tsai R J. Integrating perceived playfulness into expectation-confirmation model for web portal context[J]. Information and Management, 2005, 42(5): 683-693.
[41] Cho V, Cheng T C E, Lai W M J. The role of perceived user-interface design in continued usage intention of self-paced e-learning tools[J]. Computers and Education, 2009, 53(2): 216-227.
[42] Lee Y, Kwon O. Intimacy, familiarity and continuance intention: An extended expectation–confirmation model in web-based services[J]. Electronic Commerce Research & Applications, 2011, 10(3): 342-357.
[43] Venkatesh V, Thong J Y L, Chan F K Y, et al. Extending the two-stage information systems continuance model: Incorporating UTAUT predictors and the role of context[J]. Information Systems Journal, 2011, 21(6): 527-555.
[44] Chong Y L. Understanding mobile commerce continuance intentions: An empirical analysis of Chinese consumers[J]. Data Processor for Better Business Education, 2013, 53(4): 22-30.
[45] Wu I L. The antecedents of customer satisfaction and its link to complaint intentions in online shopping: An integration of justice, technology, and trust[J]. International Journal of Information Management, 2013, 33(1): 166-176.
[46] Akter S, Ray P, D’Ambra J. Continuance of mHealth services at the bottom of the pyramid: The roles of service quality and trust[J]. Electronic Markets, 2013, 23(1): 29-47.
[47] Limayem M, Hirt S G, Cheung C M K. How habit limits the predictive power of intention: The case of information systems continuance[J]. MIS Quarterly, 2007, 31(4): 705-737.
[48] Ajzen I. Residual effects of past on later behavior: Habituation and reasoned action perspectives[J]. Personality and Social Psychology Review, 2002, 6(2): 107-122.
[49] Venkatesh V, Thong J Y L, Chan F K Y, et al. Extending the two-stage information systems continuance model: Incorporating UTAUT predictors and the role of context[J]. Information Systems Journal, 2011, 21(6): 527-555.
[50] Escobarrodríguez T, Carvajaltrujillo E. Online purchasing tickets for low cost carriers: An application of the unified theory of acceptance and use of technology (UTAUT) model[J]. Tourism Management, 2014, 43(5): 70-88.