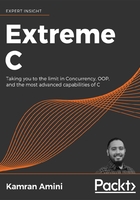
Memory management in constrained environments
There are environments in which memory is a precious resource, and it is often limited. There are also other environments in which performance is a key factor and programs should be fast, no matter how much memory we have. Regarding memory management, each of these environments requires a specific technique to overcome the memory shortage and performance degradation. First, we need to know what a constrained environment is.
A constrained environment does not necessarily have a low memory capacity. There are usually some constraints that limit the memory usage for a program. These constraints can be your customer's hard limits regarding memory usage, or it could be because of a hardware that provides the low memory capacity, or it can be because of an operating system that does not support a bigger memory (for example, MS-DOS).
Even if there are no constraints or hardware limitations, we as programmers try our best to use the least possible amount of memory and use it in an optimal way. Memory consumption is one of the key non-functional requirements in a project and should be monitored and tuned carefully.
In this section, we'll first introduce the techniques used in low memory environments for overcoming the shortage issue, and then we will talk about the memory techniques usually used in performant environments in order to boost the performance of the running programs.
Memory-constrained environments
In these environments, limited memory is always a constraint, and algorithms should be designed in a way in order to cope with memory shortages. Embedded systems with a memory size of tens to hundreds of megabytes are usually in this category. There are a few tips about memory management in such environments, but none of them work as well as having a nicely tuned algorithm. In this case, algorithms with a low memory complexity are usually used. These algorithms usually have a higher time complexity, which should be traded off with their low memory usage.
To elaborate more on this, every algorithm has specific time and memory complexities. Time complexity describes the relationship between the input size and the time that the algorithm takes to complete. Similarly, memory complexity describes the relationship between the input size and the memory that the algorithm consumes to complete its task. These complexities are usually denoted as Big-O functions, which we don't want to deal with in this section. Our discussion is qualitative, so we don't need any math to talk about memory-constrained environments.
An algorithm should ideally have a low time complexity and also a low memory complexity. In other words, having a fast algorithm consuming a low amount of memory is highly desirable, but it is unusual to have this "best of both worlds" situation. It is also unexpected to have an algorithm with high memory consumption while not performing well
Most of the time, we have a trade-off between memory and speed, which represents time. As an example, a sorting algorithm that is faster than another algorithm usually consumes more memory than the other, despite the fact that both of them do the same job.
It is a good but conservative practice, especially when writing a program, to assume that we are writing code for a memory-constrained system, even if we know that we will have more than enough memory in the final production environment. We make this assumption because we want to mitigate the risk of having too much memory consumption.
Note that the driving force behind this assumption should be controlled and adjusted based on a fairly accurate guess about the average memory availability, in terms of size, as part of the final setup. Algorithms designed for memory-constrained environments are intrinsically slower, and you should be careful about this trap.
In the upcoming sections, we will cover some techniques that can help us to collect some wasted memory or to use less memory in memory-constrained environments.
Packed structures
One of the easiest ways to use less memory is to use packed structures. Packed structures discard the memory alignment and they have a more compact memory layout for storing their fields.
Using packed structures is actually a trade-off. You consume less memory because you discard memory alignments and eventually end up with more memory read time while loading a structure variable. This will result in a slower program.
This method is simple but not recommended for all programs. For more information regarding this method, you can read the Structures section found in Chapter 1, Essential Features.
Compression
This is an effective technique, especially for programs working with a lot of textual data that should be kept inside the memory. Textual data has a high compression ratio in comparison to binary data. This technique allows a program to store the compressed form instead of the actual text data with a huge memory return.
However, saving memory is not free; since compression algorithms are CPU-bound and computation-intensive, the program would have worse performance in the end. This method is ideal for programs that keep textual data that is not required often; otherwise, a lot of compression/decompression operations are needed, and the program would be almost unusable eventually.
External data storage
Using external data storage in the forms of a network service, a cloud infrastructure, or simply a hard drive is a very common and useful technique for resolving low memory issues. Since it is usually considered that a program might be run in a limited or low memory environment, there are a lot of examples that use this method to be able to consume less memory even in environments in which enough memory is available.
This technique usually assumes that memory is not the main storage, but it acts as cache memory. Another assumption is that we cannot keep the whole data in the memory and at any moment, only a portion of data or a page of data can be loaded into the memory.
These algorithms are not directly addressing the low memory problem, but they are trying to solve another issue: slow external data storage. External data storage is always too slow in comparison to the main memory. So, the algorithms should balance the reads from the external data store and their internal memory. All database services, such as PostgreSQL and Oracle, use this technique.
In most projects, it is not very wise to design and write these algorithms from scratch because these algorithms are not that trivial and simple to write. The teams behind famous libraries such as SQLite have been fixing bugs for years.
If you need to access an external data storage such as a file, a database, or a host on the network while having a low memory footprint, there are always options out there for you.
Performant environments
As we have explained in the previous sections about the time and memory complexities of an algorithm, it is usually expected to consume more memory when you want to have a faster algorithm. In this section, we therefore expect to consume more memory for the sake of increased performance.
An intuitive example of this statement can be using a cache in order to increase the performance. Caching data means consuming more memory, but we could expect to get better performance if the cache is used properly.
But adding extra memory is not always the best way to increase performance. There are other methods that are directly or indirectly related to memory and can have a substantial impact on the performance of an algorithm. Before jumping to these methods, let's talk about caching first.
Caching
Caching is a general term for all similar techniques utilized in many parts of a computer system when two data storages with different read/write speeds are involved. For example, the CPU has a number of internal registers that perform quickly in terms of reading and writing operations. In addition, the CPU has to fetch data from the main memory, which is many times slower than its registers. A caching mechanism is needed here; otherwise, the lower speed of the main memory becomes dominant, and it hides the high computational speed of the CPU.
Working with database files is another example. Database files are usually stored on an external hard disk, which is far slower than the main memory, by orders of magnitude. Definitely, a caching mechanism is required here; otherwise, the slowest speed becomes dominant, and it determines the speed of the whole system.
Caching and the details around it deserve to have a whole dedicated chapter since there are abstract models and specific terminology that should be explained.
Using these models, one can predict how well a cache would behave and how much performance gain could be expected after introducing the cache. Here, we try to explain caching in a simple and intuitive manner.
Suppose that you have slow storage that can contain many items. You also have another fast storage, but it can contain a limited number of items. This is an obvious tradeoff. We can call the faster but smaller storage a cache. It would be reasonable if you bring items from the slow storage into the fast one and process them on the fast storage, simply because it is faster.
From time to time, you have to go to slow storage in order to bring over more items. It is obvious that you won't bring only one item over from the slow storage, as this would be very inefficient. Rather, you will bring a bucket of items into the faster storage. Usually, it is said that the items are cached into the faster storage.
Suppose that you are processing an item that requires you to load some other item from the slow storage. The first thing that comes to mind is to search for the required item inside the recently brought bucket, which is in the cache storage at the moment.
If you could find the item in the cache, there is no need to retrieve it from the slow storage, and this is called a hit. If the item is missing from the cache storage, you have to go to the slow storage and read another bucket of items into the cache memory. This is called a miss. It is clear that the more hits you observe, the more performance you get.
The preceding description can be applied to the CPU cache and the main memory. The CPU cache stores recent instructions and data read from the main memory, and the main memory is slow compared to the CPU cache memory.
In the following section, we discuss cache-friendly code, and we observe why cache-friendly code can be executed faster by the CPU.
Cache-friendly code
When the CPU is executing an instruction, it has to fetch all required data first. The data is located in the main memory at a specific address that is determined by the instruction.
The data has to be transferred to the CPU registers before any computation. But the CPU usually brings more blocks than are expected to be fetched and puts them inside its cache.
Next time, if a value is needed in the proximity of the previous address, it should exist in the cache, and the CPU can use the cache instead of the main memory, which is far faster than reading it from the main memory. As we explained in the previous section, this is a cache hit. If the address is not found in the CPU cache, it is a cache miss, and the CPU has to access the main memory to read the target address and bring required data which is quite slow. In general, higher hit rates result in faster executions.
But why does the CPU fetch the neighboring addresses (the proximity) around an address? It is because of the principle of locality. In computer systems, it is usually observed that the data located in the same neighborhood is more frequently accessed. So, the CPU behaves according to this principle and brings more data from a local reference. If an algorithm can exploit this behavior, it can be executed faster by the CPU. This is why we refer to such algorithm as a cache-friendly algorithm.
Example 5.6 demonstrates the difference between the performances of cache-friendly code and non-cache-friendly code:
#include <stdio.h> // For printf function
#include <stdlib.h> // For heap memory functions
#include <string.h> // For strcmp function
void fill(int* matrix, int rows, int columns) {
int counter = 1;
for (int i = 0; i < rows; i++) {
for (int j = 0; j < columns; j++) {
*(matrix + i * columns + j) = counter;
}
counter++;
}
}
void print_matrix(int* matrix, int rows, int columns) {
int counter = 1;
printf("Matrix:\n");
for (int i = 0; i < rows; i++) {
for (int j = 0; j < columns; j++) {
printf("%d ", *(matrix + i * columns + j));
}
printf("\n");
}
}
void print_flat(int* matrix, int rows, int columns) {
printf("Flat matrix: ");
for (int i = 0; i < (rows * columns); i++) {
printf("%d ", *(matrix + i));
}
printf("\n");
}
int friendly_sum(int* matrix, int rows, int columns) {
int sum = 0;
for (int i = 0; i < rows; i++) {
for (int j = 0; j < columns; j++) {
sum += *(matrix + i * columns + j);
}
}
return sum;
}
int not_friendly_sum(int* matrix, int rows, int columns) {
int sum = 0;
for (int j = 0; j < columns; j++) {
for (int i = 0; i < rows; i++) {
sum += *(matrix + i * columns + j);
}
}
return sum;
}
int main(int argc, char** argv) {
if (argc < 4) {
printf("Usage: %s [print|friendly-sum|not-friendly-sum] ");
printf("[number-of-rows] [number-of-columns]\n", argv[0]);
exit(1);
}
char* operation = argv[1];
int rows = atol(argv[2]);
int columns = atol(argv[3]);
int* matrix = (int*)malloc(rows * columns * sizeof(int));
fill(matrix, rows, columns);
if (strcmp(operation, "print") == 0) {
print_matrix(matrix, rows, columns);
print_flat(matrix, rows, columns);
}
else if (strcmp(operation, "friendly-sum") == 0) {
int sum = friendly_sum(matrix, rows, columns);
printf("Friendly sum: %d\n", sum);
}
else if (strcmp(operation, "not-friendly-sum") == 0) {
int sum = not_friendly_sum(matrix, rows, columns);
printf("Not friendly sum: %d\n", sum);
}
else {
printf("FATAL: Not supported operation!\n");
exit(1);
}
free(matrix);
return 0;
}
Code Box 5-12 [ExtremeC_examples_chapter5_6.c]: Example 5.6 demonstrates the performance of cache-friendly code and non-cache-friendly code
The preceding program computes and prints the sum of all elements in a matrix, but it also does more than that.
The user can pass options to this program, which alters its behavior. Suppose that we want to print a 2 by 3 matrix that is initialized by an algorithm written in the fill
function. The user has to pass the print
option with the desired number of rows and columns. Next, you can see how these options are passed to the final executable binary:
$ gcc ExtremeC_examples_chapter5_6.c -o ex5_6.out
$ ./ex5_6.out print 2 3
Matrix:
1 1 1
2 2 2
Flat matrix: 1 1 1 2 2 2
$
Shell Box 5-21: Output of example 5.6 showing a 2 by 3 matrix
The output consists of two different prints for the matrix. The first is the 2D representation of the matrix and the second is the flat representation of the same matrix. As you can see, the matrix is stored as a row-major order in memory. This means that we store it row by row. So, if something from a row is fetched by the CPU, it is probable that all of the elements in that row are fetched too. Hence, it would be better to do our summation in row-major order and not column-major order.
If you look at the code again, you can see that the summation done in the friendly_sum
function is row-major, and the summation performed in the not_friendly_sum
function is column-major. Next, we can compare the time it takes to perform the summation of a matrix with 20,000 rows and 20,000 columns. As you can see, the difference is very clear:
$ time ./ex5_6.out friendly-sum 20000 20000
Friendly sum: 1585447424
real 0m5.192s
user 0m3.142s
sys 0m1.765s
$ time ./ex5_6.out not-friendly-sum 20000 20000
Not friendly sum: 1585447424
real 0m15.372s
user 0m14.031s
sys 0m0.791s
$
Shell Box 5-22: Demonstration of the time difference between the column-major and row-major matrix summation algorithms
The difference between the measured times is about 10 seconds! The program is compiled on a macOS machine using the clang
compiler. The difference means that the same logic, using the same amount of memory, can take much longer – just by selecting a different order of accessing the matrix elements! This example clearly shows the effect of cache-friendly code.
Note:
The time
utility is available in all Unix-like operating systems. It can be used to measure the time a program takes to finish.
Before continuing to the next technique, we should talk a bit more about the allocation and deallocation cost.
Allocation and deallocation cost
Here, we want to specifically talk about the cost of Heap memory allocation and deallocation. This might be a bit of a surprise if you realize that Heap memory allocation and deallocation operations are time-and memory-consuming and are usually expensive, especially when you need to allocate and deallocate Heap memory blocks many times per second.
Unlike Stack allocation, which is relatively fast and the allocation itself requires no further memory, Heap allocation requires finding a free block of memory with enough size, and this can be costly.
There are many algorithms designed for memory allocation and deallocation, and there is always a tradeoff between the allocation and deallocation operations. If you want to allocate quickly, you have to consume more memory as part of the allocation algorithm and vice versa if you want to consume less memory you can choose to spend more time with a slower allocation.
There are memory allocators for C other than those provided by the default C standard library through the malloc
and free
functions. Some of these memory allocator libraries are ptmalloc
, tcmalloc
, Haord
, and dlmalloc
.
Going through all allocators here is beyond the scope of this chapter, but it would be good practice for you to go through them and give them a try for yourself.
What is the solution to this silent problem? It is simple: allocate and deallocate less frequently. This may seem impossible in some programs that are required to have a high rate of Heap allocations. These programs usually allocate a big block of the Heap memory and try to manage it themselves. It is like having another layer of allocation and deallocation logic (maybe simpler than implementations of malloc
and free
) on top of a big block of the Heap memory.
There is also another method, which is using memory pools. We'll briefly explain this technique before we come to the end of this chapter.
Memory pools
As we described in the previous section, memory allocation and deallocation are costly. Using a pool of preallocated fixed-size Heap memory blocks is an effective way to reduce the number of allocations and gain some performance. Each block in the pool usually has an identifier, which can be acquired through an API designed for pool management. Also, the block can be released later when it is not needed. Since the amount of allocated memory remains almost fixed, it is an excellent choice for algorithms willing to have deterministic behavior in memory-constrained environments.
Describing memory pools in further detail is beyond the scope of this book; many useful resources on this topic exist online if you wish to read more about it.