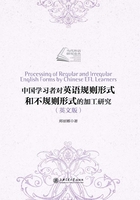
2.1 The Single-Mechanism Approach
The single-mechanism approach postulates that both regular and irregular forms in a language are processed within either a rule system or a single associative memory system using distributed representations. The theory of the former proposal is Generative Phonology (e.g., Chomsky & Halle, 1968; Halle & Mohanan, 1985; Ling & Marinov, 1993) and the representatives of the latter proposal are the connectionist models.
2.1.1 Generative Phonology
Motivated by the fact that most irregular forms are not completely arbitrary or unproductive, but rather fall into families displaying certain patterns (e.g., cling-clung, fling-flung, sling-slung, sting-stung, wring-wrung, string-strung), and that even novel forms can be generated based on existing patterns (e.g., spling-splung), Generative Phonology posits rule to explain the dichotomy of regular and irregular forms. As we see it, the regulars are generated by the rules like “adding-ed or -s”, which are further divided into different allomorphic versions depending on the phonetic environment. For Generative Phonology, irregular forms, just like regular forms, are formed by affixing an abstract morpheme to the stem and applying rule that change the stem' sphonological composition. For instance, the rule of changing i into a is applied to many irregular verbs like sing-sang, ring-rang, sit-sat, swim-swam. Thus, Generative Phonology explains the similarity between stems and their inflected forms by claiming that the rules just change a specified segment with the rest of the stems untouched in the output. The only difference between the formation of regular and irregular forms is that the rule of suffixation is applied at the end of the regular forms, while the rule of the stem vowel change is applied within the irregular forms.
Generative Phonology is actually the rule-based manipulation of symbol strings. It makes rule extremely powerful and proposes that both regulars and irregulars are processed only by a rule system. However, several potential defects for its assumptions can be noted. For one thing, although Generative Phonology captures the fact that the formation of irregular forms shows some regularity, it ignores another fact that the irregulars that are applicable to some stem vowel change rule often have some phonological similarity. For example, the rule of changing i into u is applied to a list of words cling, fling, sting, sling, swing, wring, etc whose onset is a consonant cluster and coda is ng, showing much phonological similarity. For another thing, the stem vowel change rule always have some exceptions. For example, the rule of changing i into u is applicable to cling, fling, sting, sling, swing, wring, but it fails to apply to bring, spring, ring and sing. Likewise, the rule of changing i into a is applicable to drink, sink, stink and shrink, but not think, wink or blink.
2.1.2 The Connectionist Models
Like Generative Phonology, the connectionist models also assume a single mechanism for the processing of regular and irregular forms. But different from Generative Phonology, these models claim that linguistic items are processed and represented by an associative memory, and hence both regular and irregular forms are stored and retrieved via the associative memory system.
Since the mid-1980s, a number of connectionist models have been developed to account for various psycholinguistic behavior including word recognition and morphology. One of the earliest and best known models is Rumelhart andMcClelland's (1986) model of past tense learning. Others include Seidenberg and McClelland's (1989) model of word recognition, and the models of Plunkett and Marchman (1991) and MacWhinney and Leinbach (1991). More recently, Bates and her colleagues (Bates & Goodman, 1997) proposed another connectionist model in which lexical and grammatical knowledge is subserved by a large and heterogeneous lexicon. And Plunkett and Juola's (1999) model simulated the learning of English verbal and nominal systems, taking into account common phonological patterns and showing evidence of overregularization, phonological conditioning and frequency effects. The connectionist models differ in their details, but they all posit a single pattern associatior and share the assumption that a single pattern associator memory is enough for regular and irregular forms, driven by input phonological patterns and generalization based on phonological similarity.
Connectionism offers a computational framework for the single-system approach by implementing networks that represent the mapping relationship between different word forms through associatively linked orthographic, phonological and semantic codes, by extending the mechanism of memory for the irregulars to the regulars and by challenging the need for the use of rules. Opposite to the Generative Phonology, its key idea is to make memory more powerful. In the connectionism, an item or word is not directly linked to another item or word, and rather the phonological features of an item are linked to those of another. While similar words will reinforce each other because of shared features, new words similar to the learned ones will activate the shared features and inherit the patterns that have been learned previously. In this way, the connectionist models can learn to associate features of the stem with those of its inflected form and imitate people's analogizing of the irregular patterns to new words, thus successfully acquiring the past tenses and generalizing to numerous novel verbs, even displaying overregularization errors of the irregular verbs.
As a simple illustration, let us take a close look at the working principles of connectionist models by discussing the classic connectionist model: Rumelhart and McClelland's Model of Past-tense Inflection (Rumelhart & McClelland, 1986) (see Figure 2-1). In this model, the same units and connections that produce the irregular pasttense forms from the irregular stems also process the regulars by copying the features of the stem to the pasttenses and add[t], [d]or[id]depending on the final consonants. The model consists of an array of input units and output units, and a matrix of modifiable weighted links between inputs and outputs. It is a pattern-associator network that links the relationship between the phonological forms of the stems and the pasttenses of English verbs. This network contains an encoding network on the input side and a decoding network on the output side. The network also has a series of input nodes, each representing a sound of an input stem and its environment, and an identical number of output nodes representing the past tenses. None of the links correspond exactly to a word or rule, but every input node is linked to an output node. Learning occurs in the pattern associator. The encoding network simply converts a sequence of phonemes into the “Wickelfeature” representation used inside the network to represent the stem of each word. Similarly, the decoding network converts the computed Wickelfeature representation of the attempted pasttense response back to a sequence of phonemes. Thus, a verb comes into the model by first taking it apart into its phonological features and activating the subset of input nodes corresponding to the features of the word. These nodes pass activation along the connections to the output nodes, raising their activation to different degrees. The past tense form is computed as the word that best fits the active output nodes. The activation transmitted along the connections depends on the “weights” of the connections and these connections will change gradually during a training phase. Training consists of presenting the network with stems and their correct past tenses. The connection weights change gradually to capture the correlations among stem features and past tense features. For instance, for the regular English verbs whose stems end with an unvoiced sound, the past tense will be formed by adding the unvoiced dental sound[t]. Through repeated exposure to such regular verbs, positive connections between input units and output units will be strengthened in the network and the regular past tense forms will be gradually acquired. Also, the connections between the features in the irregulars like ink and ank would be reinforced by sink-sank, drink-drank and shrink-shrank. Every pair with such a pattern will strengthen this connection weight and the trained model can generalize the pattern to new verbs like splink according to their similarity to the previously trained verbs and the connection weights. In this way, the connectionist models succeed in learning hundreds of regular and irregular verbs and generalizing to new words without representations specific to rules. Thus, in the connectionism, the inputs take on a slightly more important role as there are no special internal mechanisms containinginnate linguistic knowledge such as Universal Grammar. The learner is just like a human computer that processes linguistic information in the input. For the advocates of connectionism (e.g., Rumelhart & McClelland, 1986; Elman et al., 1996; Seidenberg, 1997), learning is the consequence of weights adjustment on connections based on statistical contingencies in the environment, and grammatical rules are nothing but descriptions of behavior.

Figure 2-1 The Rumelhart-McClelland Model of Past-tense Inflection
(Adapted from Rumelhart & McClelland, 1986: 222)
In Rumelhart and McClelland's model, there is no categorical distinction between compositional and non-compositional forms. Instead, rules are only descriptive entities and the system gradually learns the entire statistical structure of language, from the arbitrary mappingsin non-compositional forms to the rule-like mappings of compositional forms. Connectionism argues that cognitive processes should be graded, probabilistic, interactive, context-sensitive anddomain-general. The representation and computation of lexical items and grammatical rule take place over a large number of interconnected processing units. Acquisition of language and other abilities occurs via gradual adjustment of the connections among processing units. No actual rule operate in the processing of language. According to Rumelhart and McClelland (1986) and Elman et al. (1996), three key features of connectionism are gradual acquisition of the target forms, graded sensitivityto phonological and semantic content, and a single, integrated mechanism forregular and irregular forms depending jointly on phonology and semantics.
2.1.3 Summary
The two major constructs within the single-mechanism approach are Generative Phonology and the connectionist models. Their common feature is that both of them adopt the extreme position that inflectional morphology is processed and represented only by one system. But opposite to Generative Phonology which invokes only rule to generate regular and irregular forms, the connectionist models attribute inflectional morphology processing totally to an associative memory.