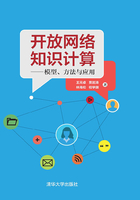
参考文献
[1] 王元卓,靳小龙,程学旗.网络大数据:现状与挑战.计算机学报,2013,36(6):1125-1138.
[2] 李国杰,程学旗.大数据研究:未来科技及经济社会发展的重大战略领域——大数据的研究现状与科学思考.中国科学院院刊,2012,27(6):647-657.
[3] Lee TB.Semantic Web architecture.XML 2000, 2000[2013-07-25].http://www.w3.org/2000/talks/1206-xml2k-tbl. 2000-11-8.
[4] Aditya P, Anand R, Hector G-M. Towards the Web of concepts: extracting concepts from large datasets. In: Proc. of the 36th Int’l Conf. on Very Large Data Bases VLDB’10. San Francisco, CA: Morgan Kaufmann Publishers, 2010. 566-577.
[5] 万长林,史忠植,胡宏,等.基于本体的语义Web服务QoS描述和发现.计算机研究与发展,2011,48(6):1059-1066.
[6] Gruber TR. A translations approach to portable ontology specifications. Knowledge Acquisition, 1993, 5 (2): 199-220.
[7] Etzioni O, Cafarella M, Downey D, et al. Unsupervised named-entity extraction from the Web: an experimental study. 2005[2013-07-25]. https://homes.cs.washington.edu/~etzioni/papers/knowitall-aij.pdf.
[8] Etzioni O, Cafarella M, Downey D, et al. Web-scale information extraction in KnowItAll: (preliminary results). In: Proc. of the 13th Int’l Conf. on World Wide Web. New York: ACM, 2004. 100-110.
[9] Banko M, Cafarella MJ, Soderland S, et al. Open information extraction from the Web. In: Proc. of the 20th Int’l Joint Conf. on Artifical Intelligence (IJCAI’07). New York: ACM, 2007. 2670-2676.
[10] Carlson A, Betteridge J, Kisiel B, et al. Toward an architecture for neverending language learning. In: Proc. of the 24th AAAI Conf. on Artifical Intelligence. Menlo Park, CA: AAAI Press, 2010. 1306-1313.
[11] Wu W, Li H, Wang H, et al. Probase: a probabilistic taxonomy for text understanding. In: Proc. of the 2012 ACM SIGMOD Int’l Conf. on Management of Data. New York: ACM, 2012. 481-492.
[12] Gallagher S.How Google and Microsoft taught search to understand the Web.2012[2013-07-25].http://arstechnica.com/information-technology/2012/06/inside-the-architecture-of-googles-knowledge-graphand-microsofts-satori/
[13] Nakashole N, Theobald M, Weikum G. Scalable knowledge harvesting with high precision and high recall. In: Proc. of the 4th ACM Int’l Conf. on Web Search and Data Mining. New York: ACM, 2011. 227-236.
[14] Suchanek FM, Sozio M, Weikum G. SOFIE: a self-organizing framework for information extraction. In: Proc. of the 18th Int’l Conf. on World Wide Web. New York: ACM, 2009. 631-640.
[15] Auer S, Bizer C, Kobilarov G, et al. DBpedia: a nucleus for a Web of open data. In: Aberer K, et al. (eds) The Semantic Web. LNCS 4825, Springer, 2007. 722-735.
[16] Biega J, Kuzey E, Suchanek FM. Inside YAGO2s: a transparent information extraction architecture. In: Proc. of the 22nd Int’l Conf. on World Wide Web. New York: ACM, 2013. 325-328.
[17] Hffart J, Suchanek F, Berberich K, et al. YAGO2: a spatially and temporally enhanced knowledge base from Wikipedia. Artificial Intelligence Journal, 2013, 194 (4): 28-61.
[18] Suchanek F, Kasneci G, Weikum G. YAGO—a core of semantic knowledge. In: Proc. of the 16th Int’l Conf. on World Wide Web. New York: ACM, 2007. 697-706.
[19] Philpot A, Hovy EH, Pantel P. Ontology and the lexicon. In: The Omega Ontology. Cambridge: Cambridge University Press, 2008. 35-78.
[20] Ponzetto S, Navigli R. Large-scale taxonomy mapping for restructuring and integrating Wikipedia. In: Proc. of the 21st Int’l Joint Conf. on Artifical Intelligence (IJCAI’09). San Francisco: Morgan Kaufmann Publishers, 2009. 2083-2088.
[21] Ponzetto S, Strube M. Taxonomy induction based on a collaboratively built knowledge repository. Artificial Intelligence, 2011, 175 (9/10): 1737-1756.
[22] 史忠植.知识发现.北京:清华大学出版社,2002.
[23] 董振东,董强,郝长伶.知网的理论发现.中文信息学报,2007,21(4):3-9.
[24] 梅立军,周强,臧路,等.知网与同义词词林的信息融合研究.中文信息学报,2005,19(1):63-70.
[25] 黄曾阳.HNC理论概要.中文信息学报,1997,11(4):11-20.
[26] 于江生,俞士汶.中文概念词典的结构.中文信息学报,2002,16(4):12-20.
[27] Lenat D,Guha R.Building large knowledge-based systems:representation and inference in the Cyc Project.Boston,MA:Addison-Wesley,1989.
[28] 许文艳,刘三阳.知识库系统的逻辑基础.计算机学报,2009,32(11):2123-2129.
[29] 钟秀琴,刘忠,丁盘苹.基于混合推理的知识库的构建及其应用研究.计算机学报,2012,35(4):761-766.
[30] 陈立玮,冯岩松,赵东岩.基于弱监督学习的海量网络数据关系提取.计算机研究与发展,2013,50(9):1825-1835.
[31] Kushmerick N, Weld D, Doorenbos R. Wrapper induction for information extraction. In: Proc. of the 15th Int’l Joint Conf. on Artifical Intelligence (IJCAI’97). New York: ACM, 1997. 1-246.
[32] Hsu C-N, Dung M-T. Generating finite-state transducers for semi-structured data extraction from the Web. Information Systems, 1998, 23 (8): 521-538.
[33] Brin S. Extracting patterns and relations from the World Wide Web. In: Proc. of the WebDB Workshop at the 6th Int’l Conf. on Extending Database Technology, EDBT’98. Berlin: Springer-Verlag, 1998. 172-183.
[34] Riloff E, Jones R. Learning dictionaries for information extraction by multi-level bootstrapping. In: Proc. of the 16th AAAI Conf. on Artifical Intelligence. Menlo Park, CA: AAAI Press, 1999. 474-479.
[35] Curran J, Murphy T, Scholz B. Minimising semantic drift with mutual exclusion bootstrapping. In: Proc. of the 10th Conf. of the Pacific Association for Computational Linguistics. Melbourne: PACLING, 2007. 172-180.
[36] Carlson A, Betteridge J, Wang R, et al. Coupled semi-supervised learning for information extraction. In: Proc. of the 3rd ACM Int’l Conf. on Web Search and Data Mining (WSDM’10). New York: ACM, 2010. 101-110.
[37] Song YQ, Wang HX, Wang ZY, et al. Short text conceptualization using a probabilistic knowledgebase. In: Proc. of the 22nd Int’l Joint Conf. on Artificial Intelligence (IJCAI’11). New York: ACM, 2011. 2330-2336.
[38] Lee T, Wang Z, Wang H, et al. Web scale taxonomy cleansing. Proc. of the VLDB Endowment, 2011, 4 (12): 1295-1306.
[39] Suchanek F, Hffart J, Kuzey E, et al. YAGO2: modular high-quality information extraction with an application to flight planning. In: Proc. of the Demo at German Computer Science Symp. , BTW2013. Magdeburg: BTW, 2013. 515-518.
[40] Lohr S.Aiming to learn as we do, a machine teaches itself.2010-10-05[2013-07-25].http://www.nytimes.com/2010/10/05/science/05compute.html?ref=global-home
[41] Kyle VH.Right now a computer is reading online, teaching itself language.Gizmodo, 2010[2013-07-25].http://www.gizmodo.com.au/2010/10/right-now-a-computer-is-reading-online-teaching-itself-language/
[42] Liu S, LiuF, Yu C, et al. An effective approach to document retrieval via utilizing WordNet and recognizing phrases. In: Proc. of the 27th Annual Int’l ACM SIGIR Conf. on Research and Development in Information Retrieval (SIGIR’04). New York: ACM, 2004. 266-272.
[43] Zamiro O, Etzioni O. Web document clustering: a feasibility demonstration. In: Proc. of the 21st Annual Int’l ACM SIGIR Conf. on Research and Development in Information Retrieval (SIGIR’98). New York: ACM, 1998. 46-54.
[44] Salton G, Yang CS, Yu CT. A theory of term importance in automatic text analysis. Journal of American Society for Information Science, 1975, 26 (1): 33-44.
[45] Broder A. A taxonomy of Web search. In: Proc. of the 25th Annual Int’l ACM SIGIR Conf. on Research and Development in Information Retrieval (SIGIR’02). New York: ACM, 2002. 3-10.
[46] Cambria E. Isanette: a common and common sense knowledge base for opinion mining. In: Proc. of the 6th Int’l Conf. on Data Mining Workshops. Piscataway, NJ: IEEE, 2011. 315-322.
[47] Wen H, Song Y-Q, Wang H-X, et al. Identifying users’topical tasks in Web search. In: Proc. of the 4th ACM Int’l Conf. on Web Search and Data Mining (WSDM’13). New York: ACM, 2013. 93-102.
[48] Guo JF. Intent-aware query similarity. In: Proc. of the 17th ACM Conf. on Information and Knowledge Management (CIKM’11). New York: ACM, 2011. 259-268.
[49] Kim D. Context-dependent conceptualization. In: Proc. of the 23rd Int’l Joint Conf. on Artifical Intelligence (IJCAI’13). Menlo Park, CA: AAAI Press, 2013. 2654-2661.
[50] 汤庸,林鹭贤,罗烨敏,等.基于自动问答系统的信息检索技术研究进展.计算机应用,2008,28(11):2745-2748.
[51] 吴友政,赵军,段湘煜,等.问答式检索技术及评测研究综述.中文信息学报,2005,19(3):1-13.
[52] Burger JC, Chaudhri V, et al.Issues, tasks and program structures to roadmap research in question answering.2001[2013-07-25].http://www.inf.ed.ac.uk/teaching/courses/tts/papers/qa_roadmap.pdf
[53] Maybury MT. New directions in question answering. Menlo Park, CA: AAAI/MIT Press, 2004.
[54] IBM.Watson.2013[2013-07-25]http://en.wikipedia.org/wiki/Watson_(computer)
[55] Kumar D, Ramakrishnan N, Helm R, et al. Algorithms for storytelling. IEEE Trans. on Knowledge and Data Engineering, 2008, 20 (6): 736-751.
[56] Hossain M, Butler P, Boedihardjo A, et al. Storytelling in entity networks to support intelligence analysts. In: Proc. of the 18th ACM SIGKDD Int’l Conf. on Knowledge Discovery and Data Mining (KDD’12). New York: ACM, 2012. 1375-1383.
[57] Zaki MJ, Ramakrishnan N. Reasoning about sets using redescription mining. In: Proc. of the 11th ACM SIGKDD Int’l Conf. on Knowledge Discovery and Data Mining (KDD’05). New York: ACM, 2005. 364-373.
[58] Fang L, Sarma AD, Yu C, et al. REX: explaining relationships between entity pairs. VLDB Endowment, 2011, 5 (3): 241-252.
[59] Quinlan JR, Cameron-Jones RM. FOIL: a midterm report. In: Proc. of the European Conf. on Machine Learning (ECML’93). Berlin: Springer-Verlag, 1993. 3-20.
[60] Mitchell TM, Betteridge J, Carlson A, et al. Populating the semantic Web by macro-reading Internet text. In: Proc. of the Int’l Semantic Web Conf. (ISWC). Berlin: Springer-Verlag, 2009. 998-1002.
[61] Cohen WW, Page D. Polynomial learnability and inductive logic programming: methods and results. New Generation Computing, 1995, 13 (34): 369-409.
[62] Lao N, Mitchell TM, Cohen WW. Random walk inference and learning in a large scale knowledge base. In: Proc. of the Conf. on Empirical Methods in Natural Language Processing (EMNLP’11). Stroudsburg, PA: Association for Computational Linguistics, 2011. 529-539.
[63] Schoenmackers S, Etzioni O, Weld D, et al. Learning first-order Horn clauses from Web text. In: Proc. of the 2010 Conf. on Empirical Methods in Natural Language Processing (EMNLP’10). Stroudsburg, PA: Association for Computational Linguistics, 2010. 1088-1098.
[64] Schoenmackers S, Etzioni O, Weld D. Scaling textual inference to the Web. In: Proc. of the Conf. on Empirical Methods in Natural Language Processing (EMNLP’08). Stroudsburg, PA: Association for Computational Linguistics, 2008. 79-88.
[65] Lao N, Cohen WW. Fast query execution for retrieval models based on path-constrained random walks. In: Proc. of the 16th ACM SIGKDD Int’l Conf. on Knowledge Discovery and Data Mining (KDD’10). New York: ACM, 2010. 881-888.
[66] Lao N, Cohen W. Relational retrieval using a combination of path-constrained random walks. Machine Learning, 2010, 81 (1): 53-67.
[67] Backstrom L, Leskovec J. Supervised random walks: predicting and recommending links in social networks. In: Proc. of the 4th ACM Int’l Conf. on Web Search and Data Mining (WSDM’11). New York: ACM, 2011. 635-644.
[68] Lichtenwalter R, Lussier JT, Chawla NV. New perspectives and methods in link prediction. In: Proc. of the 16th ACM SIGKDD Int’l Conf. on Knowledge Discovery and Data Mining (KDD’10). New York: ACM, 2010. 243-252.
[69] Adamic LA, Adar E. Friends and neighbors on the Web. Social Networks, 2001, 25 (3): 211-230.
[70] Newman M. Clustering and preferential attachment in growing networks. Physical Review E, 2001, 64 (2): 025102.
[71] Katz L. A new status index derived from sociometric analysis. Psychometrika, 1953, 18 (1): 39-43.
[72] Yin DW, Hong LJ, Davison B. Strctural link analysis and prediction in microblogs. In: Proc. of the 20th ACM Int’l Conf. on Information and Knowledge Management. New York: ACM, 2011. 1163-1168.
[73] Jia YT, Wang YZ, Li JY, et al. Structural-interaction link prediction in microblogs. In: Proc. of the 22nd Int’l Conf. on World Wide Web Companion. New York: ACM, 2013. 193-194.
[74] Liben-Nowell D, Kleinberg J. The link prediction problem for social networks. In: Proc. of the 12th ACM Int’l Conf. on Information and Knowledge Management. New York: ACM, 2003. 556-559.